The use of discrete choice experiments in applied economic analysis
Observed behaviour is usually understood to be the best indicator of future choices. This ‘revealed preference’ data is therefore favoured by practitioners as the basis for economic models. However, many economic questions involve looking at markets that do not (yet) exist, or ‘but for’ hypothetical situations that did not actually happen. How do economists approach situations where the revealed preferences of consumers are not observable?
In economics, ‘stated preference’ methods provide a way to value goods or assets that are not routinely traded on conventional markets—for example, respondents may be asked hypothetical ‘what if’ questions to assess their willingness to pay for a particular good or service.
Stated preference methods are central components of public policy analyses such as natural resource damage assessments, and are also increasingly common in transport studies and antitrust cases.1 However, stated preference surveys have been criticised for their inherent ‘hypothetical bias’, because respondents can state a value but do not have to walk the proverbial walk to pay their stated price. Such criticism has featured in several highly publicised cases such as damage assessments for the 1989 Exxon Valdez oil spill in the USA.2
Discrete choice experiments (DCEs) are an alternative stated preference technique that seek to reduce this hypothetical bias. They do so by identifying the value that respondents place on individual attributes of the hypothetical good or service. In this article we explore DCEs and some of their applications.3
What are DCEs?
The economic foundation behind DCEs is the ‘hedonic theory of demand’: this theory argues that the satisfaction that people get from buying goods and services can be better understood as the satisfaction that they get from purchasing access to the various attributes that those goods and services provide.4 For instance, in this framework a smartphone can be broken down into the quality of the camera, the brand and design, the operating system and the price. DCEs allow us to evaluate how such attributes influence the purchasing decisions of consumers.
The aim of DCEs is to help us understand the value that people place on different attributes (i.e. features) of comparable products, by assessing the decisions that they make. Because the options available to respondents are defined by the practitioner rather than observed in consumer data, DCEs allow us to evaluate hypothetical choices, for example between products that are not yet on the market.
Table 1 below shows how such a choice might be illustrated in a survey. The respondent is asked to pick their preferred option from two future hypothetical smartphone models, having considered the four different attributes. They will face repeated choice tasks, where the characteristics of each option vary slightly each time. For example, having been asked to choose between options A and B, as set out in Table 1, the respondent may then be offered another choice in which the iPhone option is more expensive than the Samsung model, but has more storage space.
Table 1 Attribute list for a smartphone
Attributes | A | B |
Brand | iPhone | Samsung |
Operating system | iOS 16 | Android 13 |
Camera | 12-megapixel | 108-megapixel |
Storage (GB) | 64 | 128 |
Price | €600 | €700 |
Source: Oxera analysis.
The survey construction is a crucial step in the process and should be guided by the underlying research question. The practitioner must decide what attributes to include, and must define the set of levels from where choice tasks are picked. For example, the pre-determined range of possible prices in this case may be €500–€1,000. The combination of attribute levels between alternatives and choice tasks is known as the survey design.
Good survey design should follow two guiding principles: the choices should be meaningful, and the attributes should be relevant—i.e. something that is in the market, or very similar to something that is in the market. Meaningful choice implies that the options are neither identical nor so skewed in favour of one option that it is always the obvious pick. Relevant attributes are those that play a role in people’s decision-making. The choices that respondents face should largely mirror choices that they face fairly regularly in their everyday life. Otherwise, respondents might not engage seriously with the different options presented to them.
Once survey data has been collected, regression analysis is used to estimate the effect of variation in each attribute on the probability of choosing a particular option.5 This allows the practitioner to value each attribute independently, and to express the ‘value’ that respondents place on a single attribute in terms of another valuation attribute (e.g. how many screen inches would be needed to compensate for the loss of one hour of battery life?).
DCEs in economic analysis
In what follows, we present three case studies—in the fields of competition economics, transport and environmental economics. These examples highlight how DCEs provide a powerful tool to estimate the value of goods, services and assets in hypothetical ‘what if’ scenarios.
DCE in antitrust litigation: BNLfood
In antitrust cases, economists often prefer to use market data rather than stated preference methods. However, when relevant market data is simply not available, DCEs based on carefully designed consumer surveys can provide valuable insights. Indeed, many antitrust cases involve the construction of a ‘but for’ counterfactual, i.e. an alternative set of market circumstances in which the alleged antitrust infringement did not occur—so engaging with hypothetical scenarios is already a familiar concept. For instance, in anticompetitive foreclosure cases, the conduct has often precluded or delayed the introduction of a new product into the market place.
Anticompetitive foreclosure was the theory of harm that BNLfood used in its 2011 antitrust litigation BNLFood Investments Limited SARL v. Martek Biosciences Corp., in the infant formula industry in the USA.6 In the 2000s, infant formula supplemented with additives became increasingly popular, thanks to its benefits for infant eye and brain development. In 2008, infant formula with additives accounted for 95% of sales, by value, in the USA. Martek had a total monopoly in the supply of supplements used in US infant formula, at least in part due to long-term exclusive supply agreements with large infant formula manufacturers. BNLfood—the complainant in the case—was a major supplier of infant formula supplements outside of the USA.
The case assessed whether Martek’s exclusive contracts were in violation of US antitrust laws.7 Economic experts on the case analysed whether Martek’s alleged anticompetitive conduct had substantially limited competition, and also the extent of damages to BNLfood. This required an estimation of the long-run market shares that BNLfood would have had in the absence of market foreclosure, i.e. assuming it was able to enter the US market (the ‘but for’ counterfactual).
In its submission to the court, BNLfood presented economic evidence based on a simple DCE targeted at recent purchasers of infant formula. Data was collected from an Internet-based survey where respondents answered a series of discrete choice tasks. It was subsequently used to estimate the relative demand for different additive types at different price points. Data from the DCE supported an estimated long-run market share of BNLfood at around 20% in the ‘but for’ counterfactual. This result showed that BNLfood’s additives appealed to some customers despite being more expansive than Martek’s ones.
DCE in transport demand forecasting: Heathrow Express
It is important for businesses to forecast demand and revenues because this partially determines the investments that they will undertake and the strategies that they will adopt. Because DCEs allow an assessment of consumer behaviour in hypothetical scenarios, they allow businesses to test the impact of different investment options. Using data collected from a DCE, Oxera forecast the demand and revenue for Heathrow Express and Heathrow Connect, which were the services providing frequent rail services between Heathrow Airport and central London’s Paddington Station.8
At the time, the accuracy of the models based on past data used for business planning was decreasing, in part because past data could not account for recent investments at Heathrow, or recent trends in the market.
Oxera therefore used a DCE to overcome these challenges and forecast consumer demand. An interesting feature of this DCE is that the data on past behaviour was used to calibrate the options shown to the respondents, so that they were as realistic as possible.
The results of this DCA allowed an assessment of customer preferences for different modes of transport, and their sensitivity to the different characteristics of the modes considered. The survey was also used to estimate the price elasticity of demand—i.e. the sensitivity of passengers to price increases—which would have been a highly complex exercise with past data. Among other things, the results of the survey shed light on the extent to which consumers valued the ‘Heathrow Express’ brand.
DCEs in environmental valuation: agri-schemes
Another application of DCEs is in environmental valuation. DCEs are commonly used in this area, either because traditional markets do not exist or because extrapolation from studies carried out in other environments is difficult. One application is estimating farmers’ willingness to participate in agri-environmental schemes that reward environmental land management (ELM).9 Under these schemes, governments provide financial support to landowners to help them make changes to their land that are beneficial to the environment.
As of 2022, three new schemes to reward ELM have been announced: (i) the sustainable farming incentive; (ii) local nature recovery; and (iii) landscape recovery. The third scheme involves the UK government providing payments to restore agricultural land through natural regeneration—‘rewilding’, or planting trees—and is planned to be offered to farmers from 2024.10 DCEs are an appropriate tool in this case, because the schemes are only hypothetical at present.
Around 300 farmers from across the north of England have so far been recruited, and compensated, to participate in an online choice experiment. Respondents were repeatedly presented with three options: two variants of potential ELM contracts, and a status quo alternative.
In the field of environmental conservation, habitat connectivity refers to the ability of individuals within a species to interact and form stable communities. This is a desirable feature of sustainable ecosystems.11 To improve habitat connectivity, a hypothetical ELM scheme was proposed, allowing contract-holders to receive a bonus for coordinating with neighbouring farmers in creating ecological corridors. Patches of retired land needed to be connected via strips of untouched land that animals could traverse safely, even if such strips crossed farm boundaries.
To evaluate the cost to farmers of providing better connectivity, a DCE was designed as illustrated in Table 2 below. The table shows two alternative contracts, A and B, where only A involves coordinating with a neighbour. This coordination requires communication and compromise, and is assumed to constitute a cost to participating farmers. As compensation, these hypothetical contracts include a £200 bonus payment to each participant.
Table 2 ELM contracts
Attributes | A | B | C |
ELM type | Planted trees | Rewilding | None |
Coordination | Yes | No | No |
Corridor width | 10m | 20m | n.a. |
Bonus | £200 | £0 | £0 |
Payment | £300 | £300 | £0 |
Note: The table is a stylised example of a choice card presented to respondents in a DCE about ELM contracts led by Durham University, UK. Respondents were presented with eight repeated choice tasks with varied attribute levels.
Source: Oxera analysis.
Preliminary data from the DCE reveals that farmers are indeed less likely to choose the option with a coordination requirement. Additional compensation is, therefore, likely to be necessary to make such schemes attractive. However, the DCE also reveals that respondents require only £4 extra in bonuses to consider a contract that requires them to collaborate with one extra neighbour. Ultimately, although farmers were, overall, unlikely to participate in the scheme without additional compensation, it was not found that it was a significant barrier to the uptake of ELM contracts.
DCEs are here to stay
DCEs are a useful tool for economists and policymakers, whether the end goal is to value non-observable environmental public goods (contingent valuation), or measure willingness to pay for goods and services in a variety of settings, from transport to competition.
Recently, competition authorities and economists have increasingly used DCEs to assess the possible effects of proposed mergers, or in defining relevant markets. For instance, in the UK Competition and Markets Authority (CMA) phase 2 investigation of the Amazon/Deliveroo acquisition, the CMA used a DCE to measure the importance of various attributes of offerings on the online convenience grocery market.12 Also, as detailed in a previous Agenda article, in the Netherlands the ACM has been using DCEs extensively in various merger investigations.13
The reason for this increasing popularity is that properly designed DCEs are a powerful alternative to surveys based on hypothetical ‘what if’ questions that are typically associated with simple stated preference methods. Even though setting up a good DCE survey design typically requires time and careful consideration, the gains (i.e. more reliable results and choice patterns) can be substantial.
1 For instance, Oxera has previously interviewed more than 1,000 homeowners to gather data on how people make decisions about energy efficiency, and undertaken a consumer survey to study consumer preferences in relation to taxi services in the UK. Oxera (2006), ‘Policies for energy efficiency in the UK household sector’, report prepared for Defra, January. Oxera (2003), ‘The consumer welfare impact of regulations on the UK licenced taxi market’, September, report prepared for the OFT (now CMA).
2 Johnston, R.J., Boyle, K.J., Adamowicz, W., Bennett, J., Brouwer, R., Cameron, T.A., Hanemann, W.M., Hanley, N., Ryan, M., Scarpa, R., Tourangeau, R. and Vossler, C.A. (2017), ‘Contemporary guidance for stated preference studies’, Journal of the Association of Environmental and Resource Economists, 4:2, pp. 319–405.
3 DCEs are sometimes known as ‘conjoint analysis’, which is a more generic marketing term. ‘DCEs’ is a more precise category with solid theoretical foundations (i.e. the random utility theory). For a technical explanation of the difference, see Louviere, J.J., Flynn, T.N. and Carson, R.T. (2010), ‘Discrete choice experiments are not conjoint analysis’, Journal of Choice Modelling, 3:3, pp. 57–72.
4 Lancaster, K. (1966), ‘A new approach to consumer theory’, Journal of Political Economy, 2, pp. 132–157.
5 Mariel, P., Hoyos, D., Meyerhoff, J., Czajkowski, M., Dekker, T., Glenk, K., Jacobsen, J.B., Liebe, U., Olsen, S.B., Sagebiel, J. and Thiene, M. (2021), Environmental valuation with discrete choice experiments: Guidance on design, implementation and data analysis, Springer Nature.
6 Akemann, M.P., Reed-Arthurs, R. and Zona, J.D. (2018), ‘Chapter 31: Conjoint analysis: applications in antitrust litigation’, in N. Mizik and D.M. Hanssens (eds), Handbook of marketing analytics: Methods and applications in marketing management, public policy, and litigation support, Edward Elgar Publishing, pp. 590–605.
7 Amended Complaint for Injunctive Relief and Damages (2011), BNLFood Investments Limited SARL v. Martek Biosciences Corp., 5 May.
8 For more details, see Jevons, D., Hoe, T. and Shepherd, M. (2011), ‘Understanding customer preferences for airport access: Implications for forecasting’, Journal of Airport Management, 5:3, pp. 226–238.
9 In collaboration with professors Ric Scarpa and Ashar Aftab at Durham University, Oxera Intern Daniel Leppert carried out a DCE studying English farmers’ willingness to participate in such schemes.
10 Department for Environment, Food & Rural Affairs (2022), ‘Environmental land management schemes: outcomes’, policy paper, 6 January (last accessed October 2022).
11 Correa Ayram, C.A., Mendoza, M.E., Etter, A. and Pérez Salicrup, D.R. (2016), ‘Habitat connectivity in biodiversity conservation: A review of recent studies and applications’, Progress in Physical Geography: Earth and Environment, 40:1, pp. 7–37.
12 Accent (2020), ‘Amazon/Deliveroo Merger inquiry research’, April, section 4 ‘Conjoint Analysis and Findings’, consulted on 24 October 2022.
13 Oxera (2017), ‘Using conjoint analysis in merger cases: a competition practitioner’s perspective’, Agenda, April.
Contact
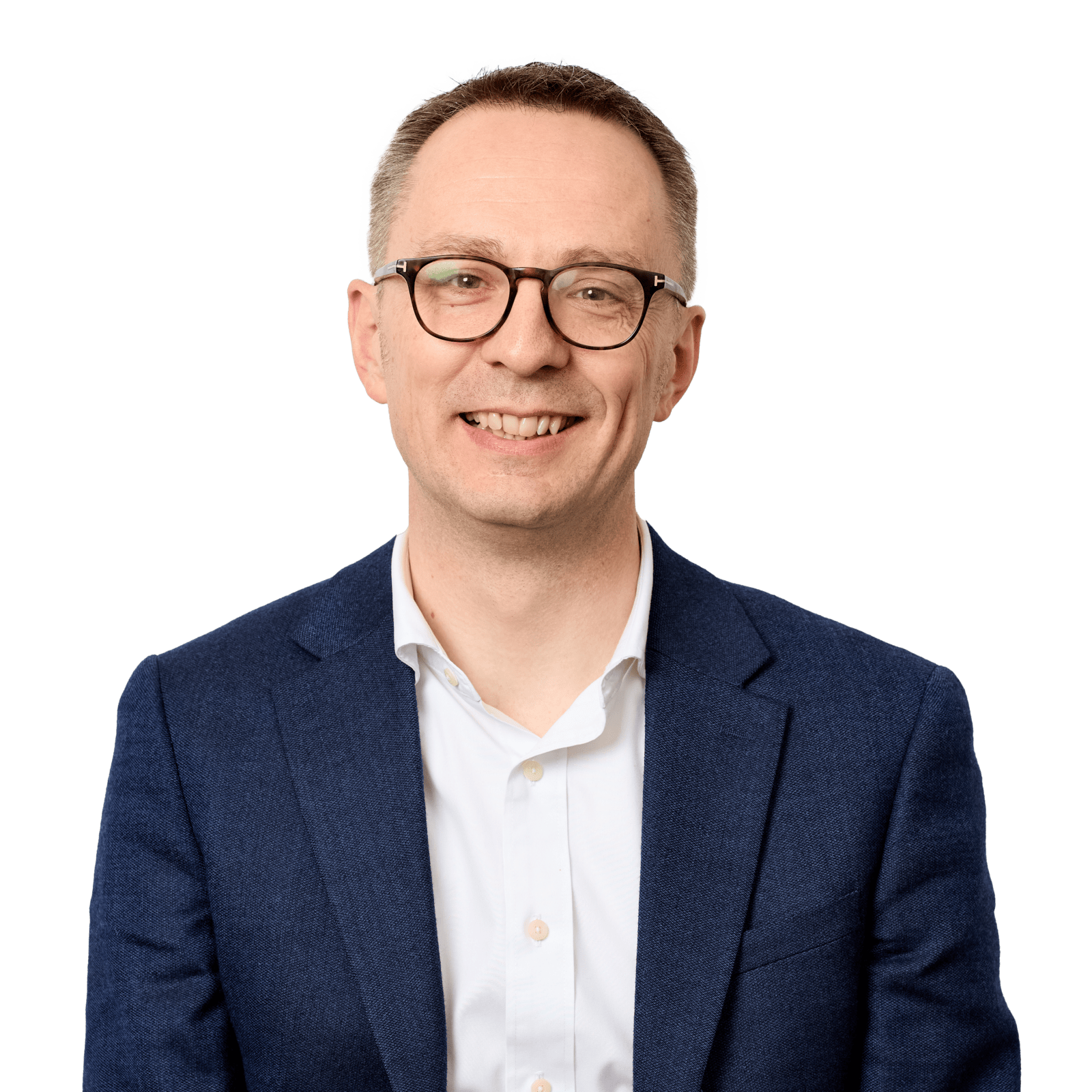
David Jevons
PartnerGuest author
Daniel Leppert
PhD candidate in Economics, Durham University, UK
Contributors
Related
Related
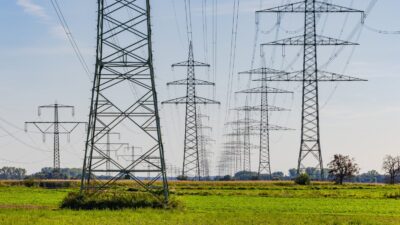
Ofgem’s RIIO-3 Sector Specific Methodology Decision
On 18 July 2024, Ofgem published its Sector Specific Methodology Decision (SSMD) for the forthcoming RIIO-3 price control period for electricity transmission (ET), gas transmission (GT) and gas distribution (GD) networks.1 This follows Ofgem’s consultation on the matter in December 2023.2 RIIO-3 will last for… Read More
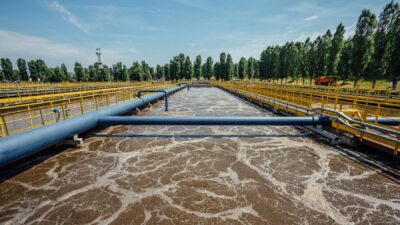
The future funding of the England & Wales water sector: Ofwat’s draft determinations
On Thursday 11 July, Ofwat (the England and Wales water regulator) published its much anticipated Draft Determinations (DDs). As part of the PR24 price review, this sets out its provisional assessment of allowed revenues and performance targets for AMP8 (2025–30)—and will be of great interest to water companies, investors,… Read More