Unreliable evidence? How (not) to use statistical significance tests
Greater availability of data and computing power has made statistics an increasingly accessible tool in regulatory investigations, policy analysis and litigation. In the majority of these applications, statistical analysis is used to estimate the impact of a specific action on market outcomes. This might include whether entry by a new supplier affects the prices of existing suppliers; whether a change in management has any effect on a firm’s efficiency; or whether a cartel had any impact on market prices.
In all cases, it is relevant to ascertain not only the size of the estimated impact, but also the reliability of the estimate. This typically involves testing for reliability from an economics perspective (i.e. whether the estimated impact makes economic sense), as well as from a statistical perspective (which usually involves deciding whether the estimated impact is sufficiently accurate to give confidence that the effect is real, and not a result of chance).
Statistical techniques can provide important information on the level of uncertainty around the estimated impact. By far the most common method is to consider whether the estimate is ‘statistically significant’.
What is statistical significance?
The aim of statistical techniques such as econometrics is to capture the ‘true’ relationships between different factors using observed data. In estimating the ‘true’ relationship—and therefore the ‘true’ impact of one factor on another—it needs to be recognised that the estimated relationship could actually be a result of a chance correlation in the data, and that the ‘true’ effect is nil. When testing for the existence of a ‘true’ effect, two types of error can occur:
- Type 1 error (false positive): the statistical estimation indicates that there is an effect, when the ‘true’ impact is zero;
- Type 2 error (false negative): the statistical estimation indicates that there is no impact, when the ‘true’ impact is present.
In essence, a statistical significance test focuses on preventing Type 1 errors. To do this, the test determines the conditional probability of an estimate at least as large as that observed, when there is actually no effect, and accepts1 the existence of an effect when this conditional probability is below a chosen threshold. Unfortunately, statistical significance tests are often misunderstood as estimating the unconditional probability of a Type 1 error. This is a subtle distinction, but has important implications for how the outcome of the test should be considered in different contexts. This issue is discussed in more detail below.
More formally, statistically significance testing is part of a branch of statistics known as ‘hypothesis testing’. The approach, largely following the work of renowned statistician, Ronald Fisher,2 involves comparing the estimated impact against a ‘ hypothesis’ that there is actually no impact and that the estimated result has been driven purely by chance (e.g. due to a certain variation in the dataset). For example, suppose that statistical estimation in a merger context indicates that the opening of a local store by one merging party, A, reduces the prices of the other merging party, B, by 1%. While this is plausible according to economic theory (which posits that more local competition leads to lower prices), it may be that the new store of A is actually too different (e.g. in terms of products or quality of service offered) to have any effect on B’s prices, and that the 1% price impact is driven purely by the specific nuance of the dataset. In other words, there is a false positive outcome.
Fisher’s approach tests for the conditional probability of a false positive—i.e. the probability that the data would produce a result that is at least as large as the estimated impact when there is, in truth, no underlying relationship between the two factors. This probability is the ‘p-value’.
Using the merger example, if the p-value for the estimated price impact is found to be 0.5 then, if there were no real effect, a result at least as large as that observed would occur 50% of the time. On the other hand, if the p-value is 0.01, this implies that, if there were no real effect, there is only a 1% chance that normal variations in the price charged by firm B would have resulted in a finding at least as large as that observed. All else being equal, the lower the p-value, the lower the probability that the estimated impact simply reflects normal variations in the underlying data. However, the p-value does not estimate the actual probability that the estimated impact simply reflects normal variations in the underlying data—this is because the test calculates the probability that the observed effect would occur on the assumption that there was no real effect. This is a common misconception, and is known as the ‘p-value fallacy’.
Despite this issue in interpretation, Fisher’s statistical significance approach remains the benchmark for assessing the reliability of estimated effects in the majority of disciplines that use statistical analysis.3 The obvious questions are then: how low is low enough? What should be the threshold for the p-value below which the estimated relationship would be considered reliable?
Setting the bar
In principle, there is no fixed threshold below which an estimate is considered to be a reliable representation of a true underlying relationship—i.e. where the estimated relationship is ‘statistically significant’. This is because the choice of the threshold depends on the extent to which the risk of ‘errors’ can be tolerated given the problem at hand.
The level adopted as the threshold for the p-value reflects the conditional error rate for Type 1 errors—i.e. when there is no effect, how frequently the practitioner will mistakenly say that there is an effect. Also, given a threshold for the p-value, the procedure implicitly determines the conditional probability of a Type 2 error.
Hence, choosing the threshold presents a dilemma to practitioners: a stringent test with a low threshold runs the risk of rejecting a genuine effect (false negative), while a lenient test with a high threshold may cause random fluctuations in the data to be mistaken for a real effect (false positive).
Fisher’s personal preference was to use 5% as a threshold for the p-value. However, its subsequent adoption by the majority of statisticians is only a convention and other thresholds, particularly 1% and 10%, are also commonly used by practitioners (but are also only conventions). Adopting these thresholds in all cases does not consider their impact on the ability of the procedure to identify real effects.
In general, the only way to improve the performance of the testing procedure is to obtain more data. As such, the appropriate threshold should be chosen depending on the context of the data available, the question being studied, and the reason for the study. This is because, in contrast to the situation envisaged by Fisher, much analysis must work within the constraints of the data that already exists. For example, when investigating the impact of a cartel or other competition law infringement, limitations and practicalities of record-keeping generally fix the amount of data that can be employed. Analysis may also be undertaken as part of a commercial decision-making process or policy evaluation with real budget considerations. In such cases the analysis does not seek to answer the philosophical question of whether an effect exists; rather, the focus is on determining the best estimate of an effect, given the data available.
If Fisher’s method is employed in these cases, the approach will effectively be to say that there is an effect only when the estimated size of the effect is sufficiently large—and often, this is not the intention of the analysis.4 In other words, it is sometimes necessary to fit the testing procedure around the data available, rather than to gather data to fit the testing procedure. This can mean moving the threshold in either direction. All else being equal, as the data available for study increases, the ability of the testing procedure to correctly identify effects that are present also increases. In many instances, particularly with the ever-increasing sizes of the datasets available for study, it may be reasonable to impose a threshold that is lower (and thus more stringent) than 5%. In other cases, where the dataset is small or ‘noisy’, the threshold may reasonably be higher.
There are no objective rules about how to make these decisions. An appropriate threshold needs to be chosen on a case-by-case basis, balancing the relative risks of the types of error that could be made in the context of that piece of analysis, and the subsequent costs and benefits.
Balancing the risks
As discussed above, there are two types of error in statistical significance testing: false positives and false negatives. The properties of statistical testing procedures with respect to these error types are defined conditionally:
- if there is an effect, what is the probability of identifying it and thereby avoiding false negatives (technically known as the power of the test)?
- if there is no effect, what is the probability of mistakenly saying that there is and thereby reaching a false positive (technically known as the size of the test)?
In general, the only way to improve both of these at the same time is to use more data. As discussed above, this is often not practical. In some cases, the power of the test can be improved without increasing the size of the dataset, by carefully structuring the test to account only for the effects that might conceivably exist. For example, when testing the estimated effect of a cartel on prices, the test could be restricted to identifying effects greater than zero, rather than all those effects that are different from zero (that is, to ignore the possibility that the cartel lowered prices). This would increase the power of the test without increasing the size of the dataset.
As a rule, however, the question of interest is not the conditional probability of the error types, but the inference that can be made on the basis of particular results. The correct inference can be quite different from the levels implied by the size and power of the test. For example, suppose that a financial economist has devised a test—for whether a stock is subject to insider trading—which is 90% accurate when an infringement has taken place, but also spuriously indicates insider trading in 5% of cases when no infringement has taken place. That is, the test has a size of 5% and a power of 90%. On this basis, the test appears to be fairly accurate. Now suppose that 1% of stocks are actually subject to insider trading—how likely is it that an infringement actually took place when the test indicates it did? The answer is surprisingly low, at 15.3%.5 This indicates a high risk of a false positive. Conversely, the likelihood that insider trading has taken place when the test indicates no infringement (a false negative) is extremely low, at 0.01%.6
Using these probabilities, a financial regulator could make informed decisions about what course of action to follow on the basis of the outcome of the test. The problem is that the authority does not know the proportion of stocks (1% in the above example) in which insider trading has occurred in the first place.
A ‘Bayesian’ statistician would say that the financial regulator should be able to get a good idea of the probability of insider trading from market research or economic theory. Bayesian statisticians approach statistical testing with quantified prior beliefs about the likelihood of particular outcomes (e.g. from previous studies or theoretical predictions), and update those beliefs based on the data available. This is in contrast to ‘frequentist’ statisticians (including Fisher), who believe that having a quantified prior belief of a probability does not make sense—either insider trading took place in a specific case, or it did not.
Bayesian approaches are not commonly used in hypothesis testing, but the principles can help decision-makers understand how to treat the outcome of statistical significance tests. In particular, in cases where outside evidence suggests that a particular effect is highly likely to occur, but the statistical significance does not meet conventional academic standards, caution should be exercised in rejecting the finding. Conversely, if an effect is highly unlikely, a prudent decision-maker should bear in mind the risk of false positives when assessing the result of statistical tests based on conventional standards of significance.
Conclusion
The methods commonly used by statisticians, including economists, to test whether results are statistically significant do not provide estimates of whether a conclusion is right or wrong. Conventional statistical significance testing using a 5% threshold is a rule of thumb that places varying evidential standards on the results of analysis, and does not consider what the purpose of the analysis is in the first place—i.e. whether it is to provide the best possible estimate of the effect, or to test for the existence of the effect (and, if so, to what standard).
Practitioners using statistics for decision-making purposes should base decisions on the strength of the evidence, including information that is external to the specific dataset available. A balanced review of the available evidence on the size and likelihood of effects, the costs and benefits of different options, and the relative risks of false positives and negatives, is usually the key to making the right judgement.
1 Technically, ‘does not reject’.
2 For example, see Fisher, R.A. (1925), Statistical Methods for Research Workers, Oliver and Boyd.
3 Although one journal, Epidemiology, did attempt to ban them from the articles that it published.
4 In extreme cases, the only effects that might be considered statistically significant, given a particular level of variability in the data, might lie entirely outside the reasonable range that the effects could take.
5 The 15.3% figure is calculated as follows: 1% of stocks are insider-traded, of which 90% are accurately identified, giving 0.9% of the total stocks. 99% of stocks are not insider-traded, but in 5% of these cases the test erroneously suggests that they are—i.e. 4.95% of stocks. In total, then, 0.9/(0.9+4.95) = 15.3% of stocks that test positively have actually been insider-traded.
6 The 0.01% figure is calculated as follows: 1% of stocks are insider-traded, of which 10% are erroneously identified as not being insider-traded—i.e. 0.1% of total stocks. 99% of stocks are not insider-traded, of which 95% are correctly identified as not being insider-traded—i.e. 94.05% of all stocks. In total, then, 0.1/(0.1+94.05) = 0.01%.
Download
Related
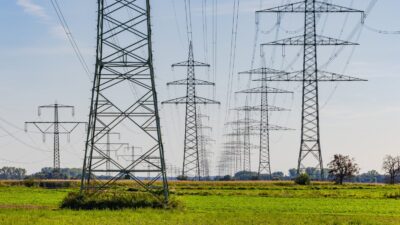
Ofgem’s RIIO-3 Sector Specific Methodology Decision
On 18 July 2024, Ofgem published its Sector Specific Methodology Decision (SSMD) for the forthcoming RIIO-3 price control period for electricity transmission (ET), gas transmission (GT) and gas distribution (GD) networks.1 This follows Ofgem’s consultation on the matter in December 2023.2 RIIO-3 will last for… Read More
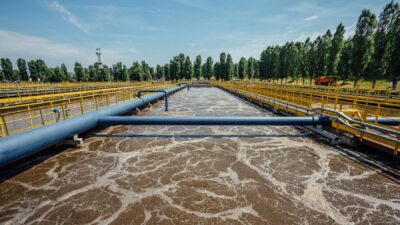
The future funding of the England & Wales water sector: Ofwat’s draft determinations
On Thursday 11 July, Ofwat (the England and Wales water regulator) published its much anticipated Draft Determinations (DDs). As part of the PR24 price review, this sets out its provisional assessment of allowed revenues and performance targets for AMP8 (2025–30)—and will be of great interest to water companies, investors,… Read More