Understanding and influencing behaviour: economics vs science
Have you heard the terms behavioural science and behavioural economics floating around? Individuals, corporations and governments are turning to both in the quest to understand and change human behaviour—but does their application add value? And what is the difference between these two similarly named disciplines? With reference to our own experience utilising both in real-world situations, Oxera is weighing in on the debate.
Behavioural science and behavioural economics are two fields that have seen a significant rise in mainstream popularity in recent years, arguably catalysed by the publication of Nudge, by Richard Thaler and Cass Sunstein, in 2009.1 Following its release, studies of human behaviour have had increasing influence over government and company policies.2 Yet the often interchangeable use of the two terms continues to create confusion. Does this matter?
The reality is that behavioural economics and behavioural science are different fields with their own theories and methodologies. However, both can be highly impactful in understanding the behaviour of participants in markets and society. This knowledge can then be applied to a range of problems—from helping regulators to encourage innovation to increasing the proportion of the population who are registered as organ donors.
Looking over the academic literature and online articles, there is an apparent inability to reach a consensus over true definitions of and distinctions between the two fields. Members of Oxera’s behavioural practice also struggled to come up with perfect definitions, but a debate on the topic resulted in some key ideas and differences being identified:
Definitions:
- Behavioural science draws on multiple disciplines, such as psychology, sociology and anthropology, to study and understand human behaviour as a whole.
- Behavioural economics incorporates lessons from other social sciences, mainly psychology, and applies them to economic problems.
Quantitative approach:
- Behavioural science concepts are applied via data science techniques to create behavioural data science, which deciphers real data to predict future behaviour.
- Behavioural economics utilises trials from experimental economics to test economic theories and behavioural biases by examining causality between variables.
Our thoughts on which longer-established fields contribute most to the discussed subfields is portrayed below in Figure 1.
Figure 1 Discipline overlaps
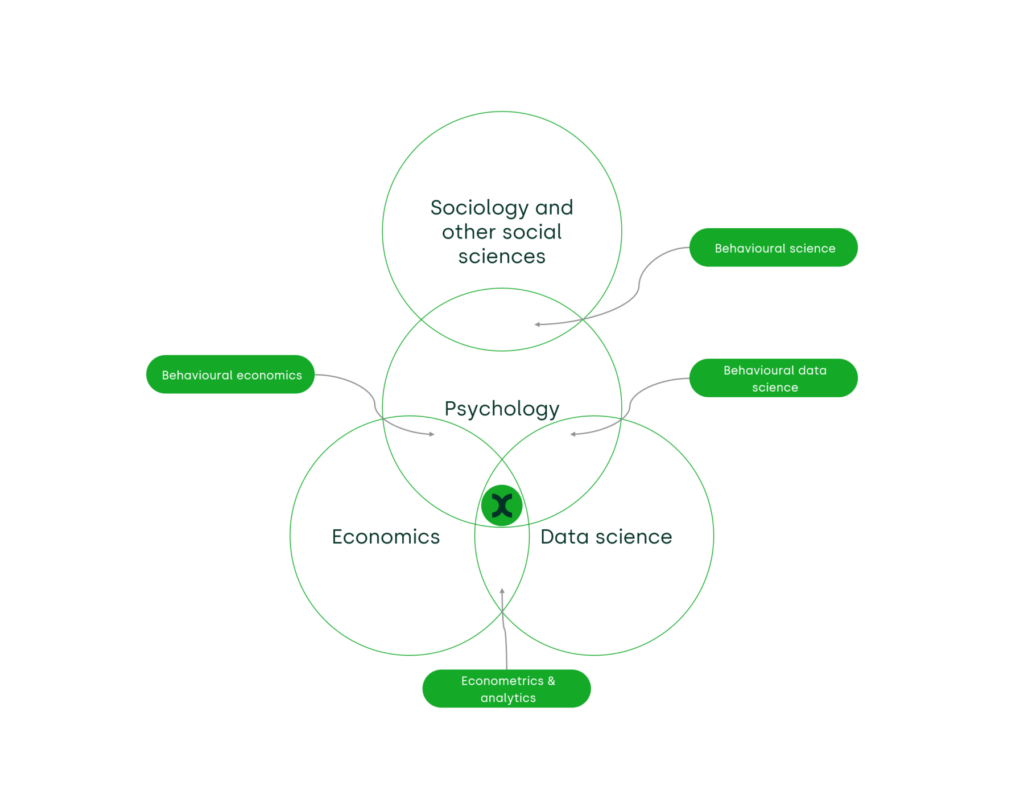
Source: Oxera.
Heads: behavioural science
Behavioural science provides a conceptual framework based on ideas and theories from a range of disciplines, with the aim of understanding why people make the decisions they do, when they do.
When applying these theories to real-world problems, we often turn to behavioural data science approaches. We typically begin by building or acquiring a dataset relevant to the issue at hand. Through the use of data science and analytics, we can begin to categorise the data by individual qualities and demographics, and then predict their behaviours. These predictions can then be used to inform people and organisations on the potential strategies that can lead to better decision-making.
One such example can be found in our report for The Investing and Saving Alliance (TISA)—which investigated some approaches to encourage more people to invest into Stocks and Shares Individual Savings Accounts (S&S ISAs) in the UK.3 Part of the analysis required a data science approach in order to segment the country’s population based on their investing behaviours, as demonstrated below in Figure 2.
Figure 2 Segmentation of UK population based on investing behaviours
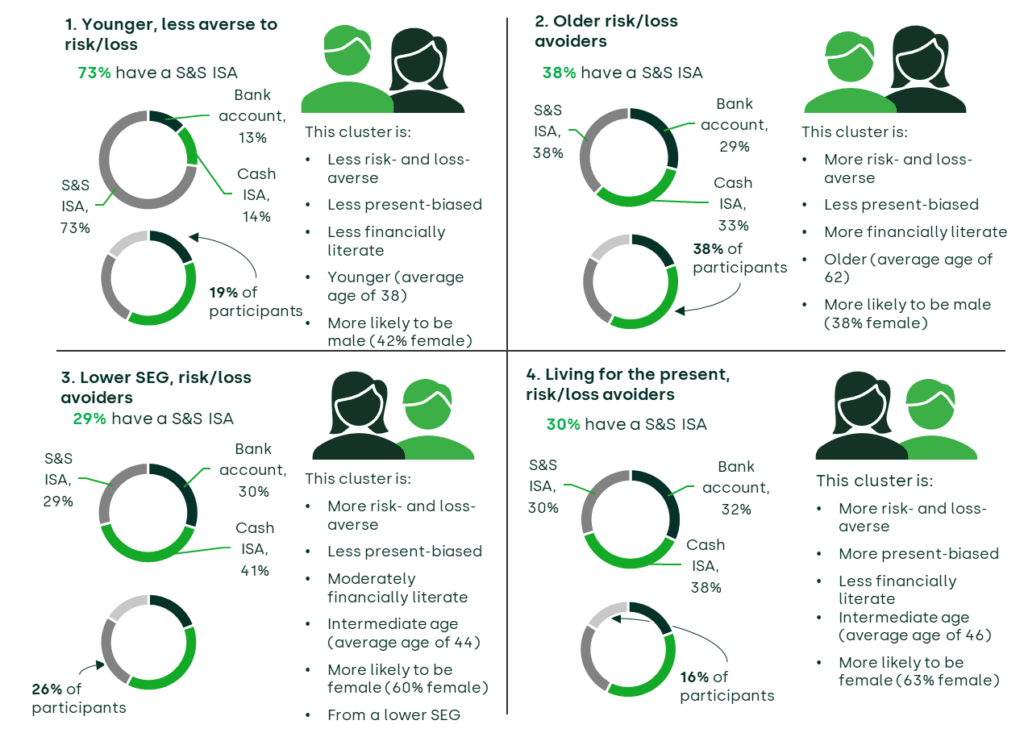
Source: Oxera.
Carrying out this clustering analysis provided us with an understanding of an individual’s propensity to invest in an S&S ISA, based on several characteristics. This allows us to predict, for example, that a risk-averse middle-aged woman from a lower socio-economic group (SEG) is less likely to have an S&S ISA than a younger, less risk-averse man. This, in turn, can ensure that any interventions are relevant and targeted. In this example, more inclusive marketing and consumer communications could result in people from the former group feeling more knowledgeable and confident to set up an S&S ISA.
The application of data science to understand behaviour is not limited to individuals—it can also be applied to the behaviour of organisations. Such an approach was taken to gauge how life insurers were adhering to the Financial Conduct Authority’s (FCA) Consumer Duty regulations regarding terms and conditions (T&Cs).4
This was done through the use of natural language processing (NLP) using the programming language Python.5 NLP gives computers the ability to understand text and spoken word in much the same way as humans can.6 Oxera developed such an algorithm that was able to determine the time it would take to read the T&Cs, and calculate the reading age required to do so. These were then compared with the reading time and age required for articles published in The Sun and Financial Times. The outcomes for both are shown in Figures 3 and 4, respectively.
Figure 3 Time required to read articles and T&Cs (hours)
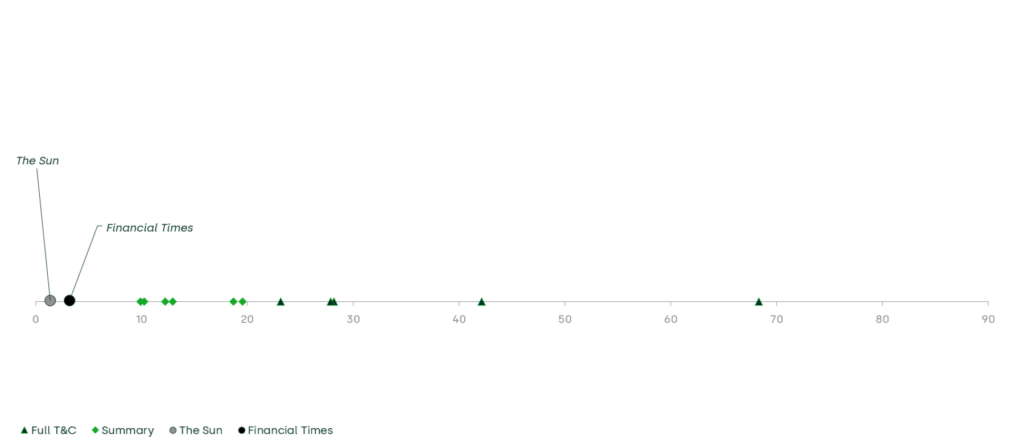
Source: Oxera.
Figure 4 Reading age required to read articles and T&Cs
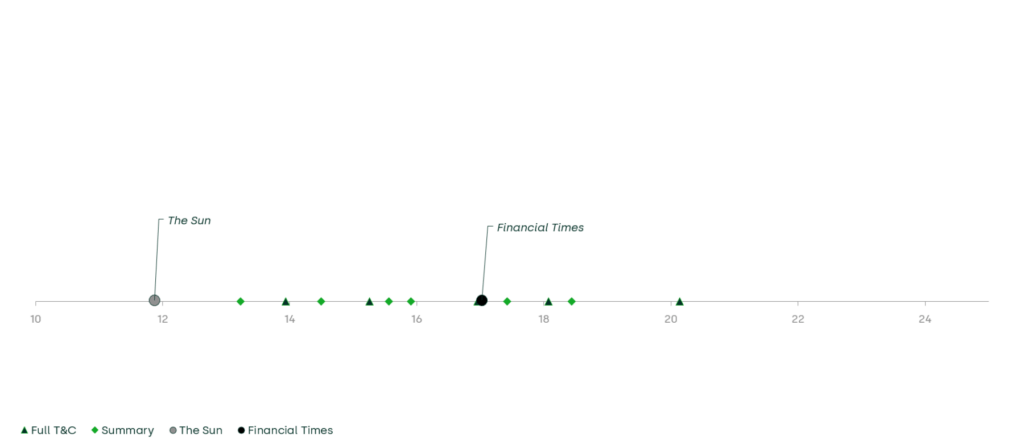
Source: Oxera.
As demonstrated in Figure 3 and Figure 4, articles from The Sun (one of the most-read daily papers in the UK) take significantly less time to read, and require a lower reading ability than T&Cs for life insurance products. Furthermore, the necessary reading age for some life insurance products is above that of the Financial Times, requiring a reading age of between the high teens and early twenties. Considering that one in seven UK adults have literacy skills at or below that of a nine- to 11-year old, this indicates that the insurance firms may not be abiding by the Consumer Duty’s requirement to ‘equip customers with the right information, at the right time, to understand the product or service in question’.7
Data science techniques like this help to demonstrate behaviours, such as those by the insurance firms, and predict behaviours, like the expectation for many consumers to be unable or unwilling to read the full T&Cs for life insurance products.
Utilising data science’s capabilities to understand behaviours gives us the ability to identify areas where outcomes are sub-optimal and prescribe appropriate remedies. In the example of insurance firms above, we can predict that firms are not making T&Cs digestible for the average consumer, perhaps giving the insurers the opportunity to take advantage of their lack of knowledge and sell them unessential products.
Tails: behavioural economics
Behavioural economics is rooted in economic theory, but also refers to psychology to better understand (and predict) individuals’ behaviours, as well as the strategic behaviour of firms and market outcomes. It usually stems from a hypothesis based on traditional economic theory, which appears to be disproven by behaviour in reality.
The notion of rationality is crucial to traditional economic models, both in terms of preferences and behaviours. Models aggregate individual preferences and then consider the average consumer as if they had complete information. If this were the case, economic agents would have transitive, stable and complete preferences, and they would behave in a way that maximised their utility—after a full and objective consideration of the costs and benefits of alternative courses of action. Yet we know that for humans this is not the case.8
First, our preferences may differ to those assumed by traditional economics. Loss aversion is a cognitive bias that explains why people typically feel more pain when losing something as compared with the pleasure experienced from gain. While loss aversion is a natural evolutionary trait (to protect one’s lot, however defined), it can also be taken advantage of by firms. In addition, our preferences may not be consistent over time (e.g. I may know that it is in my interests to take out a pension, but such decisions may be continually put off to another day—‘present bias’). While some may be aware of their such self-control problems and commit to avoiding them, others may be less aware—resulting in poor decision-making.
Second, we have cognitive limitations in making decisions. To provide a more accurate description of human problem-solving capabilities, behavioural economists coined the term ‘bounded rationality’. Since an individual’s brainpower and time are limited, humans cannot be expected to solve difficult problems ‘optimally’; rather, people adopt heuristics (rules of thumb) as a way to use their effort and time efficiently.9 While such shortcuts may save time, they do not always result in optimal outcomes—especially when firms, governments and other institutions may seek to take advantage of our natural behaviours.
Through consideration of biases, psychology, and the observed outcomes of human choices, we are able to better understand the real causes of such irrational preferences and behaviours—although we may never attain a complete understanding. It is also important to analyse and predict how firms adapt their behaviour to the existing biases, and how they affect market outcomes and consumer welfare.
One such observed outcome is present in the UK annuities market, with an annuity being a financial product that allows retirees to use their pension pot to purchase the security of a fixed income stream for their remaining years.
Oxera and the Nuffield Centre of Experimental Social Sciences (CESS) carried out a randomised controlled trial (RCT) in an online lab with a sample of 55–65 year olds.10 Participants were required to navigate a series of decisions on their retirement sources. Crucially, different prompts were provided once they reached the stage of selecting an annuity. Each prompt (besides the control) attempted to encourage the participant to shop around rather than simply purchasing an annuity from their existing pension provider, something that 60% of consumers had been shown to do.11
Through the combined usage of behavioural economic ideas and experimental economic trials, it was identified which nudges and demographic characteristics were most influential in increasing the likelihood of desired consumer behaviours. The most effective prompt gave participants a personalised message, showing the extra annual income they could gain if they purchased their annuity through a different provider, as displayed in Figure 5. This made them around three times more likely to shop around, showing the effectiveness of behavioural influence on economic decisions.
Figure 5 Personalised prompt
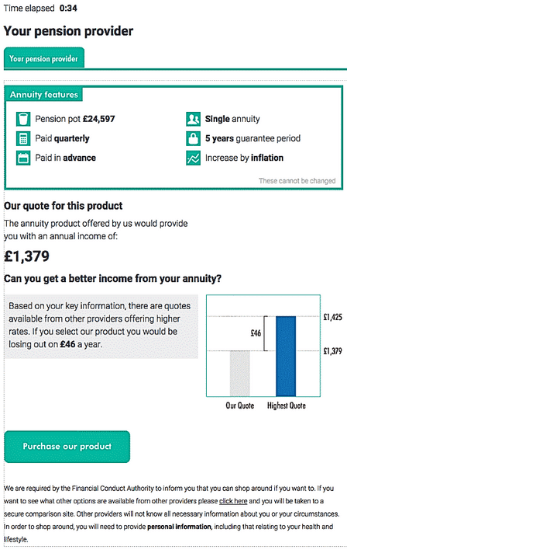
Source: Oxera.
Following pension reforms in 2015, British adults have increased flexibility with their pension pots and are no longer forced to purchase an annuity.12 The result has been the decreased popularity of this product, in favour of alternatives such as income drawdown and cash withdrawals. However, the above study is still useful in understanding which prompts are more effective in influencing consumer behaviour.
Through such experiments, we can find links between choice architecture, how options are presented, and the economic decisions made by an individual. The question for policymakers is how to intervene to help consumers make better choices.
There is some controversy here. Often, the implementation of such findings applies ‘Libertarian paternalism’, the idea that behaviour can be influenced through nudges and incentives, but the individual’s freedom of choice is never infringed upon. Although the likes of Sunstein and Thaler advocate this, nudge theory is not the sole approach to addressing behavioural inefficiencies, with Figure 6 covering the entire spectrum of policies. For example, nudges alone may have their limits, and there may be a need to ban certain practices by firms (or consumers).13 We will return to the issue of firms employing ‘dark patterns’ to catch consumers out below.
Figure 6 Policy spectrum to deal with behavioural issues
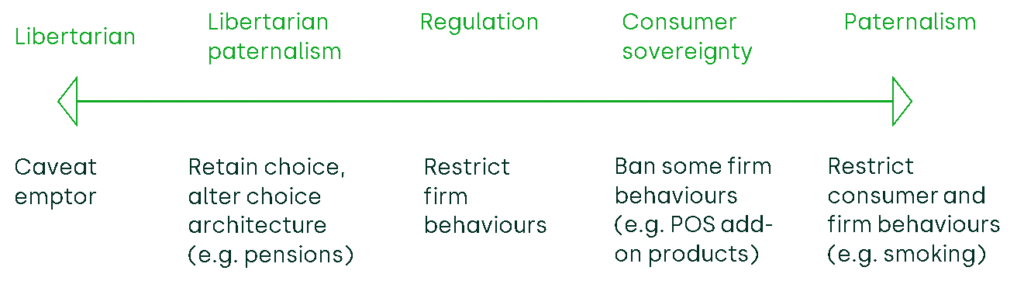
Source: Oxera.
As organisations are simply large collections of people, the presence of irrationality can be applied to firms, as Oxera found in our contribution to The Behavioral Economics Guide 2022.14 Assumed to follow profit-maximising behaviour so that they will survive in the long term, evidence shows that many firms are sustainable without profit-maximising. Assessing firm behaviour to inform policy decisions is an excellent use of behavioural economic theory. Here, Industrial Organisation (IO) modelling can be deployed, as shown in Figure 7 below.
Figure 7 How IO models can be used to assess firm behaviour
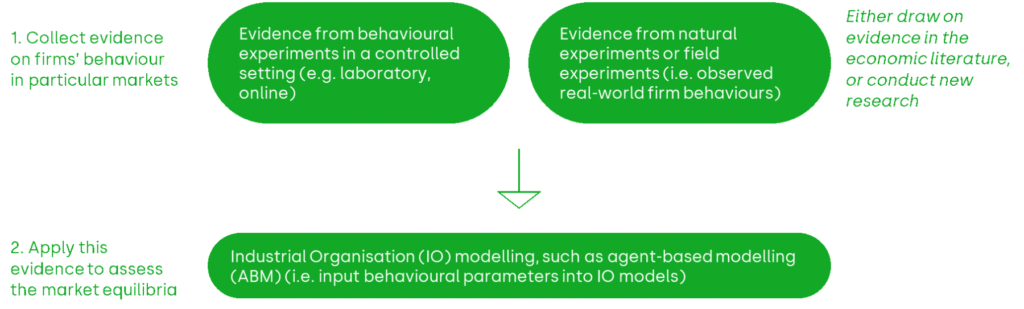
Source: Oxera.
Oxera took such an approach when we were asked to conduct an experiment for Amazon on the impact of regulatory inventions, such as the proposed EU Digital Markets Act (DMA), on innovation in the EU.15 Students at Universität Wien, Austria, were tasked with acting as ‘global’ and ‘local’ firms, and making investment decisions in different regulatory circumstances.
The findings demonstrated the potential unintended consequences of regulations proposed to benefit local firms by holding back global competitors. Due to the resulting reduction in competitive pressures, there was a significant fall in innovative efforts by the local players. With competition being one of the key drivers of innovation, this appears to be a rational response.
This is an example of noticing the occurrence of an undesirable action—in this case, local firms failing to innovate—and utilising behavioural economics to understand the causes for this phenomenon. In turn, this assists Oxera (as advisers) and the regulator (as the policy implementer) to consider potential consequences of their regulations that are highlighted through an understanding of behaviour.
Another area where regulators and competition authorities actively refer to the insights of behavioural and experimental economics is the prevalence of the deceptive practices employed by firms online (also referred to as ‘dark patterns’). These practices result from the existence of human biases, but they may also amplify them. Behavioural economics serves to generate the prediction of how these biases might affect competition and market outcomes, while experimental economics is used to measure the potential impacts and test the effectiveness of various policy responses.16
Complements or substitutes?
Are behavioural science and behavioural economics complementary fields, or can they be substituted at will to answer questions related to human behaviour?
Although the case studies provided above predominantly utilised only one of the disciplines, we believe the use of behavioural science and behavioural economics is complementary. The inefficiencies of rigid theoretical models is part of what led to the development of behavioural studies. Why be bound by certain theories or fields? Ultimately, it is the problem that dictates where we look for the optimal response. By utilising all the best models at our disposal, we strive to provide the most actionable insights.
Through the predictive powers of data science and behavioural science, we can identify and predict behaviours by certain individuals, and through a behavioural economics lens, we can understand how biases can impact economic decision-making and what can be done to influence this in an optimal way.
Both are highly valuable disciplines with similar names—but different applications. Whether you are a regulator, a company, or an individual, it is beneficial to better understand the behaviours (and their causes) of economic actors.
1 Thaler, R. H. and Sunstein, C. R. (2009), Nudge: Improving decisions about health, wealth and happiness, Penguin.
2 Fusaro, R., Sperling-Magro, J. (2021), ‘Much anew about “nudging”’, McKinsey & Company, 6 August.
3 Oxera (2022), ‘The keys to unlocking greater investment in Stocks and Shares ISAs’.
4 Oxera (2022), ‘Interminable: who can read T&Cs?’, Agenda, October.
5 See Oxera (2022), ‘Interminable: who can read T&Cs?’, Agenda, October.
6 IBM, ‘What is natural Language Processing (NLP)’.
7 Financial Conduct Authority (2022), ‘Finalised Guidance: FG22/5 Final non-Handbook Guidance for firms on the Consumer Duty’, p. 72.
8 See Oxera (2017), ‘The science of misbehaving@ Richard Thaler wins the Nobel Prize’, Agenda, October.
9 Simon, H.A. (1955), ‘A behavioral model of rational choice’, The Quarterly Journal of Economics, 69:1, pp. 99–118. Conlisk, J. (1996), ‘Why bounded rationality?’, Journal of Economic Literature, 34:2, pp. 669–700.
10 Oxera and the Nuffield Centre for Experimental Social Sciences (2016), ‘Increasing consumer engagement in the annuities market: can prompts raise shopping around?’.
11 Financial Conduct Authority (2014), ‘Thematic Review of Annuities’, February.
12 HM Revenue & Customs (2015), ‘Pension changes 2015’, 6 April.
13 For further reading on examples of this, see Oxera (2020), ‘COVID-19: behavioural economics in the spotlight’, Agenda, May.
14 Hogg, T., Fields, L. and Firullo, C. (2022), ‘Applying Behavioural Economics to Firms’, in A. Samson (ed.), The Behavioral Economics Guide 2022, pp. 89–97.
15 Oxera (2021), ‘The Digital Markets Act and incentives to innovate’, May.
16 To learn more about the economics of dark patterns, see Oxera (2021), ‘Bits of advice: the true colours of dark patterns’, Agenda, November; Barros, L., Klein, T., Shchepetova, A. and Hogg, T. (2022), ‘The rise of dark patterns: does competition law make it any brighter?’, Competition Law Journal, 21:3, pp. 136–144.
Related
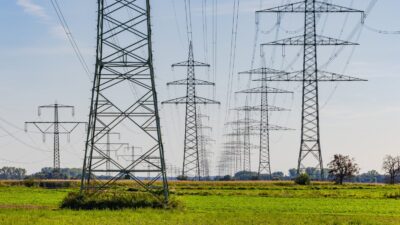
Ofgem’s RIIO-3 Sector Specific Methodology Decision
On 18 July 2024, Ofgem published its Sector Specific Methodology Decision (SSMD) for the forthcoming RIIO-3 price control period for electricity transmission (ET), gas transmission (GT) and gas distribution (GD) networks.1 This follows Ofgem’s consultation on the matter in December 2023.2 RIIO-3 will last for… Read More
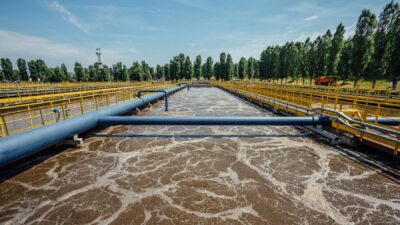
The future funding of the England & Wales water sector: Ofwat’s draft determinations
On Thursday 11 July, Ofwat (the England and Wales water regulator) published its much anticipated Draft Determinations (DDs). As part of the PR24 price review, this sets out its provisional assessment of allowed revenues and performance targets for AMP8 (2025–30)—and will be of great interest to water companies, investors,… Read More