The risks of using algorithms in business: artificial price collusion
Increasingly, prices are set by algorithms rather than humans. Many competition authorities have voiced their concerns that this may enable firms (knowingly or otherwise) to avoid competitive pressure and collude. Exactly how would such algorithmic collusion work? And what can businesses and other organisations that use pricing algorithms expect from competition authorities in the future?
In this third article on the economic consequences of algorithms and the associated risks to businesses,1 we look at the rise of pricing algorithms. How do pricing algorithms benefit competition, and how does algorithmic collusion work? How suitable are the current legal tools in dealing with algorithmic collusion? And what do businesses and other organisations using pricing algorithms need to do in response to increased regulatory vigilance?
In 2017, a Danish artificial intelligence company, a2i systems, started offering its services to petrol station operators within Germany. The products that it offers ‘allow [petrol stations] to rapidly and intelligently react to changing customer behaviour, changing markets, and unexpected events’.2 The product proved to be very successful; it is estimated that by mid-2018, the adoption rate of automated algorithmic pricing software by German petrol stations increased to around 30%, the majority of which coincided with the well-publicised market entry of a2i systems.3
On the one hand, these pricing algorithms enable businesses to set their prices more efficiently and effectively, reducing costs and increasing market efficiency. However, many competition authorities have voiced their concerns that pricing algorithms, such as those offered by a2i systems, may help firms to avoid competitive pressures and (knowingly or otherwise) coordinate with their competitors. Competition authorities are showing an increased willingness to act on this concern, and many authorities have already published studies on the topic.4 Moreover, in a press release concerning its proposed new market investigation tool,5 the European Commission explicitly cited ‘algorithm-based technical solutions’ and their propensity for tacit collusion as a potential subject for investigation. Given these conflicting perspectives on algorithmic pricing, how might we respond intelligently to its increased use?
The pro-competitive effects of pricing algorithms
Let’s start with the positive. As with many more familiar business practices—such as information exchange, asset sharing or vertical restrictions—algorithmic pricing offers many pro-competitive and efficiency-enhancing effects alongside the potential risks.6 There are at least three ways in which pricing algorithms can produce win-win outcomes for both firms and their consumers.
Cost reductions
It can be difficult for a multi-product firm to identify the ‘right’ price for all of its products—and this is particularly challenging for online retailers that sell hundreds or even thousands of different products in a fluctuating market with changing costs and inventories. Here, the use of automated decision rules or optimisation algorithms when setting prices can lead to significant efficiency gains. These cost savings can then, in whole or in part, be passed on to consumers through lower prices.
Optimal price discovery
Well-functioning markets are powerful mechanisms for allocating scarce resources, so long as prices are set ‘just right’. If prices are too high, there will be too few consumers willing to buy; if prices are too low, there will be too few producers willing to sell.
Pricing algorithms can help competitive markets function better by improving this overall price discovery process. Using data analytics, pricing algorithms can enable firms to more quickly identify the optimal price—especially in rapidly changing market conditions. Not only will this help the market to find an equilibrium of buyers and sellers, but it will signal where entrepreneurs should focus their resources and efforts to provide the products most valued by consumers.
Reduced barriers to entry
Pricing algorithms may also help firms to enter new markets previously reserved for knowledgeable and experienced players. For example, the marketing and pricing of toys previously required good knowledge of what children like and the latest playground trends, typically built on years of experience. However, with the introduction of online pricing algorithms, manufacturers can now let the data do the work for them, automatically experimenting with different prices for different toys—starting with a small assortment and gradually expanding based on actual sales.
This ability to enter unknown markets and be guided by self-generated data analytics can help level the playing field between new firms and established incumbents. Similarly, existing retailers may find it easier to broaden their product offering and include products about which they may have less expertise.
What are the concerns?
Despite the many potential pro-competitive justifications for the use of pricing algorithms, there is a concern that pricing algorithms may—inadvertently or otherwise—lead to anticompetitive market outcomes. For instance, pricing algorithms may lead to unwanted forms of price discrimination7 or increased barriers to entry when reliant on proprietary data.8 Furthermore, as we discuss below, the use of pricing algorithms could lead to another prominent concern: algorithmic collusion.
At a high level, it is possible to identify at least four different ways in which pricing algorithms may lead to collusion—each with varying degrees of feasibility in practice.9
I. Explicit algorithmic collusion
A 2017 EU e-commerce sector inquiry shows that a majority of online retailers use algorithms to monitor competitor prices, with approximately two-thirds using algorithms to automatically adjust prices in response.10
The increasing ubiquity of automated pricing can, however, make it easier for competing managers with malicious intent to implement a price agreement. Rather than having to continuously discuss and calibrate joint pricing behaviour, they can now use simple algorithms instead.
The prominent example is the 2016 GB-Eye Trod case in the UK (known as the 2015 Topkins case in the USA), in which competing online poster sellers were charged for using pre-programmed pricing algorithms to coordinate prices in a differentiated and unstable market.11 This is, of course, just as illegal as conventional cartel arrangements contrived in smoke-filled rooms. The key difference, however, is that the algorithm made the implementation and monitoring of the agreement far more straightforward.
II. Algorithmic hub-and-spoke collusion
A second way in which pricing algorithms can undermine competition is through a ‘hub-and-spoke’ construction. Here, a common supplier (the ‘hub’) coordinates the prices of downstream competitors (the ‘spokes’), without the need for these downstream competitors to formulate a horizontal agreement among themselves.
While illegal, building a solid case around allegations of hub-and-spoke collusion is generally more difficult than explicit horizontal collusion—as it requires proof that the downstream ‘spokes’ that are competing with each other are aware of the likely collusive consequences when giving up their pricing autonomy.12
The UK Competition and Markets Authority has already voiced concerns of algorithmic hub-and-spoke collusion in the context of third-party pricing software providers—their concern being that a dominant pricing software provider in an industry may act upon its ability and incentive to deploy algorithms that take into account the pricing spillovers of competitors—effectively orchestrating collusion.13
A specific allegation of digital hub-and-spoke collusion was voiced in a 2016 US class action against Uber, which alleged that Uber acted as a hub in a hub-and-spoke conspiracy by orchestrating the prices of its drivers through its common surge-pricing algorithm.14 The class action against Uber was eventually dismissed on the grounds that Uber competes with transport more generally, including public transport.
It is important to note that there is to date no empirical evidence that the use of third-party pricing software providers leads to collusive outcomes. Notwithstanding this, the increased use of vertical relations in algorithmic price-setting does raise clear theoretical concerns about the ability and incentive of firms to coordinate prices.15 The fact that this coordination occurs via a vertical channel raises the concern that the line between an illegal explicit cartel and legal tacit collusion may become much more blurred.
Platform operators and pricing software firms that supply to competing firms are therefore likely to receive increased scrutiny for their role in the price-setting behaviour of competing businesses.
III. Tacit algorithmic collusion
Collusion may not always be explicit. Pricing algorithms may also enable firms to unilaterally implement strategies that have the effect of preventing aggressive pricing in the market—in effect, reaching a tacit collusive outcome that is nearly impossible to prosecute.
However, reaching a stable but silent understanding on high prices is not easy. Firms have different cost structures and inventories, and new firms may enter the market and demand may fluctuate—factors that destabilise a tacit understanding to keep prices high.
At the same time, the practical feasibility of tacit human collusion because of algorithms should not be discounted. As RepricerExpress, a leading e-commerce pricing software supplier, communicates:16
Instead of worrying so much about having the lowest costs among your competitors, RepricerExpress recommends avoiding a price war as a technique for coming out on top. […] Within RepricerExpress, there are features to help sellers detect and avoid a price war.
For a competition authority, any ambition to ‘avoid a price war’ may sound like an attempt to collectively maintain high prices and is accordingly a potential concern—even if it is achieved tacitly and via an automated process.17
Moreover, pricing algorithms may be specified in ways that unwittingly lead to higher prices. For instance, recent academic research has shown that when competing algorithms fail to properly account for each other’s prices, which is often the case, they may underestimate their own price elasticity—the downward response in demand for their own product(s) when they increase prices.18 The net effect is that firms set prices too high.
Other academic research has shown that when competing algorithms have similar perceptions of what the optimal price points are, they may end up experimenting with equivalent prices. This, in turn, may cause them to see higher prices as optimal, not knowing that it is because they have managed to reach a supra-competitive coordinated outcome.19
While such learning specifications might be regarded as irrational or suboptimal, and not technically collusion, their use may still be explained by current limitations in what pricing algorithms can or cannot do in practice.
IV. Autonomous algorithmic collusion
The biggest concern may arise, however, when algorithms can learn to optimally form cartels all by themselves—not through instructions from their human masters (or some irrational behaviour), but through optimal autonomous learning (i.e. ‘self-learning’ algorithms). Such an outcome, were it to occur, may be very difficult to prosecute, as businesses deploying such algorithms may not even be aware of what strategy the algorithm has learned.
The big question, though, is how practicable such autonomous algorithmic collusion is in practice. Two recent academic papers have shown that such autonomous collusion is, in principle, feasible.20 The research is based on computer simulation experiments in which competing firms learn to set optimal prices using reinforcement learning—i.e. where the algorithm learns through independent trial-and-error exploration. Both papers find that the firms indeed learn collusive strategies in which they keep prices high to match their competitors, and only undercut and compete if their competitors do so.
However, many practical limitations for such autonomous algorithmic collusion remain—such as the need for a long learning period in a stable market environment. However, these papers show that autonomous algorithmic collusion is, at least in principle, possible. Moreover, advances in artificial intelligence may be able to deal with these practical limitations sooner than we might expect.
Old wine in new bottles?
Examining concerns around algorithmic collusion raises questions regarding practical feasibility and the apparent suitability of the current enforcement framework—something that is still often overlooked.
For instance, when discussing the GB-Eye Trod posters case, it is often quickly pointed out that this is ‘just old wine in new bottles’: this was a standard price-fixing cartel between competing sellers, but implemented using simple rules-based pricing algorithms.21 The existing Article 101 TFEU antitrust framework is suitable to deal with such cases, and the case has been successfully prosecuted in the UK and USA based on incriminating email correspondence. However, the key point is that managers may succumb to the temptation of such a form of algorithmic collusion much earlier, as it is relatively easy to implement—and increasingly so, with the growing availability of off-the-shelf pricing software.
Conversely, when discussing concerns around tacit algorithmic collusion or autonomous algorithmic collusion, competition authorities may find it very difficult to build a case in the absence of explicit communication or proof of a ‘meeting of minds’ by the firms involved.22 However, in such cases it should also be recognised that it is much more difficult to successfully reach a stable collusive outcome.
Figure 1 provides a stylised illustration of this general tension between practical feasibility and enforcement. This tension may offer authorities some degree of comfort: either algorithmic collusion concerns can be tackled using existing legal and compliance tools, or else the concerns are less likely to occur in practice.
However, it should be noted that this relationship is not static—and we turn to this matter next.
Figure 1 Tension between practical feasibility and enforcement concerns
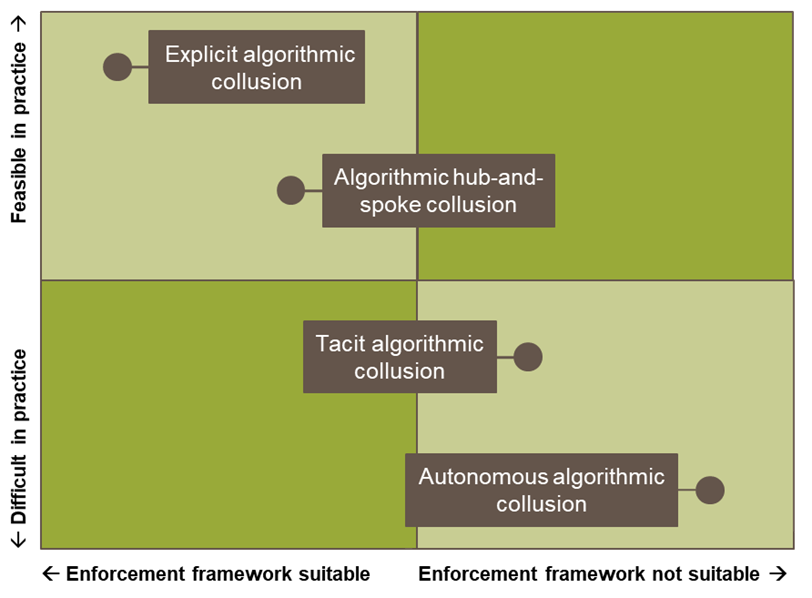
AI antitrust on the move
Businesses and other organisations using pricing algorithms—or keen to explore their potential—must be increasingly aware of any anticompetitive consequences that may result from their use of pricing algorithms. This need is driven by two forces.
- Technological advances—developments in computer science and artificial intelligence are making novel types of anticompetitive conduct increasingly feasible.
- Increased regulatory vigilance—digital conduct previously unnoticed is now increasingly on the radar of authorities that are both willing and able to act.
The first force—technological advances—pushes the dots in Figure 1 upwards. While previously even explicit human collusion using algorithms was difficult due to the absence of off-the-shelf pricing algorithms, this is no longer the case. Increasingly, businesses are relying on pricing software, possibly supplied by a common pricing software provider, raising concerns about the incentive and ability for hub-and-spoke collusion. And while autonomous algorithmic collusion is still only shown in computer simulations, its practical limitations may soon be dealt with by novel advances in artificial intelligence.
Furthermore, the second force—increased regulatory vigilance—pushes the dots to the right. Business may no longer find comfort in the fact that even if their conduct has an anticompetitive effect, enforcement may be too difficult to pursue.
Anticipating regulatory vigilance
So what can businesses expect from authorities? First, machine learning tools can similarly be used to detect cases of collusion.23 For instance, the French Competition Authority recently created a digital economy unit to develop these competencies (in the same way as several other authorities).24
Second, the use of pricing algorithms by firms will be increasingly scrutinised or even audited. As John Moore, Etienne Pfister, and Henri Piffaut (the last two of whom are the Chief Economist and Vice-President at the French Competition Authority respectively) recently proposed:25
[…] firms could be required […] first to test their algorithms prior to deployment in real market conditions (‘risk assessment’), then to monitor the consequences of deployment (‘harm identification’).
Moreover, the US Deputy Assistant Attorney General for Criminal Enforcement, Richard Powers, recently stated:26
Just as there’s a role for corporate compliance programs in deterring price fixing that occurs in traditional smoke-filled rooms, there’s a role for corporate compliance programs in preventing collusion effectuated by algorithms.
This echoes earlier statements by EU Competition Commissioner Margrethe Vestager, who remarked on the ‘need to make it very clear that companies can’t escape responsibility for collusion by hiding behind a computer program’.27
Pricing algorithms have great potential for the promotion of competition—they can reduce costs, increase market efficiency, and promote market entry. These benefits can apply to a markets as diverse as petrol pricing, airline tickets, e-commerce, and financial market trading.
However, this does not mean that the authorities have no need for concern and vigilance. There are legitimate concerns regarding competition. On the German retail petrol market, a recent academic working paper shows that the rise of pricing algorithms has led to reduced competition and increased margins—up to 28% for areas where two competing petrol stations both adopted algorithmic pricing.28 The study highlights that it is a strictly economic assessment and does not pass any legal judgment on whether there is anticompetitive behaviour—but results like these will attract the attention of authorities and regulators.
Overall, the benefits that pricing algorithms can provide to firms and their customers are desirable. When pursuing these benefits, businesses and other organisations using pricing algorithms need to also reflect on the competition concerns involved—so that they can show that they are indeed getting their margin on the merit.
1 The first two articles are Oxera (2020), ‘The risks of using algorithms in business: demystifying AI’, Agenda in focus, September; and Oxera (2020), ‘The risks of using algorithms in business: artificial intelligence and real discrimination’, Agenda in focus, October.
2 A2isystems.com (2020), ‘PriceCast technology’.
3 Assad, S., Clark, R., Ershov, D. and Xu, L. (2020), ‘Algorithmic Pricing and Competition: Empirical Evidence from the German Retail Gasoline Market’, CESifo Working Paper no. 8521, August.
4 See in particular OECD (2017), ‘Algorithms and Collusion: Competition Policy in the Digital Age’, September; UK Competition and Markets Authority (2018), ‘Pricing Algorithms: Economic working paper on the use of algorithms to facilitate collusion and personalised pricing’, 8 October; Autoridade da Concurrência (2019), ‘Digital Ecosystems, Big Data and Algorithms’; and Autorité de la Concurrence and Bundeskartellamt (2019), ‘Algorithms and Competition’, November.
5 European Commission (2020), ‘Antitrust: Commission consults stakeholders on a possible new competition tool’, press release, 2 June.
6 See also Oxera (2017), ‘When algorithms set prices: winners and losers’, 19 June.
7 UK Competition and Markets Authority (2018), ‘Pricing Algorithms: Economic working paper on the use of algorithms to facilitate collusion and personalised pricing’, 8 October.
8 Autorité de la Concurrence and Bundeskartellamt (2019), ‘Algorithms and Competition’, November; and OECD (2016), ‘Big Data: Bringing Competition Policy to the Digital Era’, November.
9 The categorisation here can be seen to build on the ‘messenger’, ‘hub-and-spoke’, ‘predictable agent’, and ‘digital eye’ categorisation in Ezrachi, A. and Stucke, M. E. (2017), ‘Artificial Intelligence & Collusion: When Computers Inhibit Competition’, University of Illinois Law Review.
10 European Commission (2017), ‘Final report on the E-commerce Sector Inquiry’, 10 May.
11 United States Department of Justice (2015), ‘Former E-Commerce Executive Charged with Price Fixing in the Antitrust Division’s First Online Marketplace Prosecution’, press release, 6 April.
12 OECD (2019), ‘Roundtable on Hub-and-Spoke Arrangements – Background Note’, 3−4 December.
13 Ibid. footnote 7.
14 Spencer Meyer v Travis Kalanick, 15 Civ 9796; 2016 US. Dist. Lexis 43944.
15 Harrington, J. E. (2020), ‘Third Party Pricing Algorithms and the Intensity of Competition’, unpublished working paper.
16 Repricerexpress.com (2020), ‘How to Avoid a Price War on Amazon’.
17 Autoridade da Concurrência (2019), ‘Digital Ecosystems, Big Data and Algorithms’.
18 Cooper, W. L., Homem-de-Mello, T. and Kleywegt, A. J. (2015), ‘Learning and Pricing with Models that do not Explicitly Incorporate Competition’, Operations Research, 63:1, pp. 86−103.
19 Hansen, K., Misra, K., and Pai, M. (2020), ‘Algorithmic Collusion: Supra-Competitive Prices via Independent Algorithms’, CEPR Discussion Paper No. DP14372; Svitak, J. and Van der Noll, R. (2019) ‘De mechanismes van algorithmische collusie’, Tijdschrift van Toezicht, 3.
20 Klein, T. (2020), ‘Autonomous Algorithmic Collusion: Q-Learning Under Sequential Competition’, Amsterdam Center for Law & Economics Working Paper No. 2018-05; and Calvano, E., Calzolari, G., Denicolo, V., and Pastorello, S. (2020) ‘Artificial Intelligence, Algorithmic Pricing, and Collusion’, American Economic Review, 110:10, pp. 3267−97.
21 See, for instance, Li, S. and Xie, C. C. (2018), ‘Automated Pricing Algorithms and Collusion: A Brave New World or Old Wine in New Bottles?’ The Antitrust Source, December; and Schrepel, T. (2020) ‘The Fundamental Unimportance of Algorithmic Collusion for Antitrust Law’, Harvard Journal of Law and Technology, February.
22 See, for instance, Ezrachi, A. and Stucke, M. E. (2017), ‘Artificial Intelligence & Collusion: When Computers Inhibit Competition’, University of Illinois Law Review; and Harrington, J. E. (2018), ‘Developing competition law for collusion by autonomous artificial agents’, Journal of Competition Law & Economics, 14:3, pp. 331−63.
23 Huber, M. and Imhof, D. (2019), ‘Machine learning with screens for detecting bid-rigging cartels’, International Journal of Industrial Organization, 65, pp. 277−301.
24 Autorité de la Concurrence (2020), ‘The Autorité de la concurrence announces its priorities for 2020’.
25 Moore, J., Pfister, E. and Piffaut, H. (2020), ‘Some Reflections on Algorithms, Tacit Collusion, and the Regulatory Framework’, Antitrust Chronicle, July, pp. 14−20.
26 MLex (2020), ‘Algorithms pose increasing antitrust compliance risk, warn US, EU officials’, 16 September.
27 Vestager, M. (2017), ‘Algorithms and Competition,’ Speech at the Bundeskartellamt 18th Conference on Competition, Berlin.
28 Ibid. footnote 3.
Download
Related
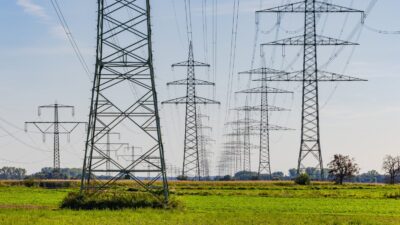
Ofgem’s RIIO-3 Sector Specific Methodology Decision
On 18 July 2024, Ofgem published its Sector Specific Methodology Decision (SSMD) for the forthcoming RIIO-3 price control period for electricity transmission (ET), gas transmission (GT) and gas distribution (GD) networks.1 This follows Ofgem’s consultation on the matter in December 2023.2 RIIO-3 will last for… Read More
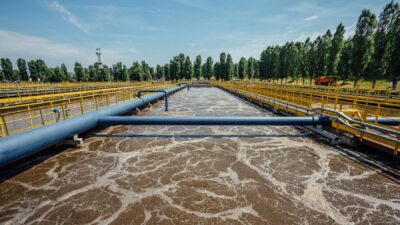
The future funding of the England & Wales water sector: Ofwat’s draft determinations
On Thursday 11 July, Ofwat (the England and Wales water regulator) published its much anticipated Draft Determinations (DDs). As part of the PR24 price review, this sets out its provisional assessment of allowed revenues and performance targets for AMP8 (2025–30)—and will be of great interest to water companies, investors,… Read More