System failure: a problem for behavioural economists?
Recent developments in neuroscience show that decision-making is more complex than the (basic) model of System 1 and System 2 that was formulated for use in behavioural economics by the Nobel Prize-winning psychologist Daniel Kahneman. Recognising the substantial impact of the discipline on public policy and regulation, Peter Andrews—Oxera Senior Adviser and former Chief Economist at the UK Financial Conduct Authority—considers the implications of Kahneman’s model and the emerging critique of behavioural economics. He shows that behavioural economists’ empirical work does not, in fact, rely on the model, and flags the success of many behavioural interventions. Insights from neuroscience are found to have improved the design and impacts of behavioural remedies.
Kahneman’s best-selling book Thinking, Fast and Slow describes in detail the model of System 1 and System 2 thinking. We are offered the following precis:1
[Kahneman] explains the two systems that drive the way we think and make choices. One system [1] is fast, intuitive, and emotional; the other [2] is slower, more deliberative, and more logical. Kahneman exposes the extraordinary capabilities—and the faults and biases—of fast thinking, and reveals the pervasive influence of intuitive impressions on our thoughts and behavior.
Figure 1 provides a summary of the two systems (with the heuristics, or ‘rules of thumb’ shortcuts, that we use on a day-to-day basis arguably falling between the two systems).
Figure 1 The two-system model
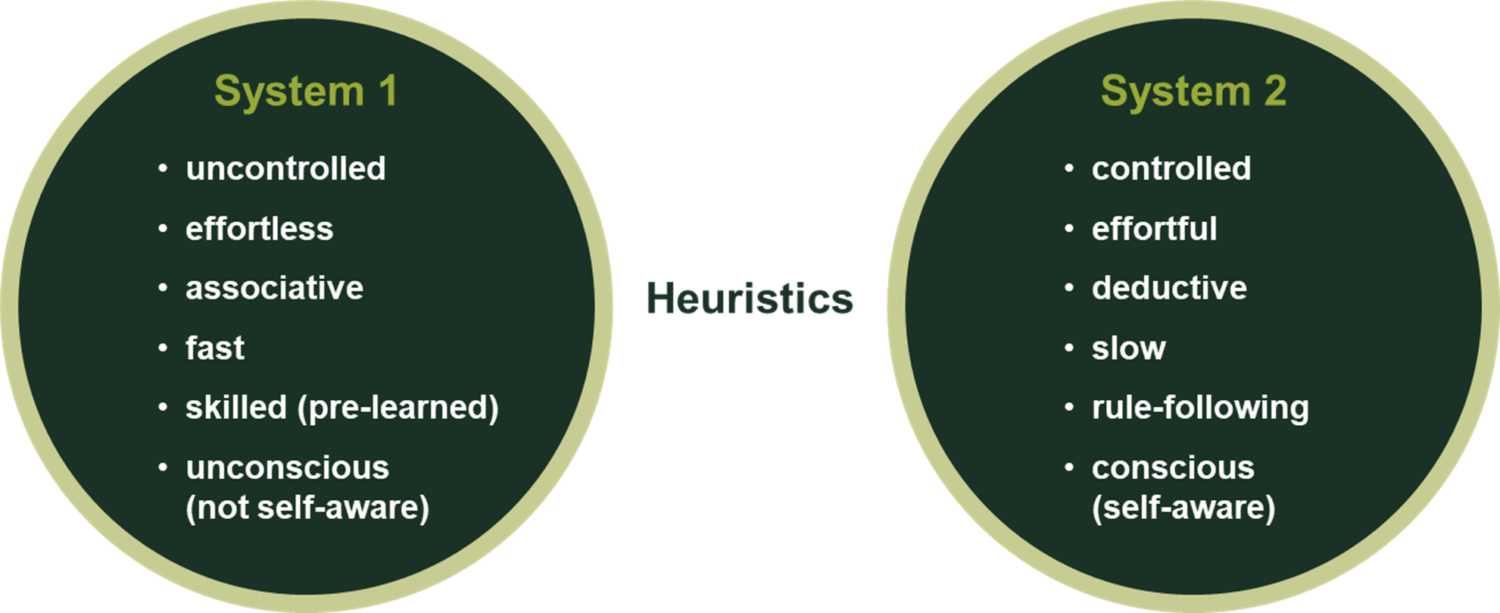
Thinking, Fast and Slow, and the work underpinning it, had a substantial effect on the practice of economics, at least as used in the development of public policy.2 This effect is understandable. As Mullainathan and Thaler explain, ‘The standard economic model of human behaviour includes three unrealistic traits—unbounded rationality, unbounded willpower, and unbounded selfishness—all of which behavioural economics modifies’.3 There are clearly some contexts in which the real-world absence of these traits matters from a public interest perspective. One example is under-saving for retirement.4
What if the two-system model is wrong?
The standard economic model of human behaviour, as described above, involves an extreme case of System 2 thinking, with the agent in possession of perfect information identifying the necessary calculations, executing them perfectly, and acting on the results. Thus the critical contribution of Thinking, Fast and Slow to the development of behavioural economics is the introduction of the concept of System 1 thinking and its distinction from System 2. In turn, the contribution of behavioural economics to economics as a whole is the introduction of the two-system model.
Yet recent empirical work suggests that the System 1–System 2 model may be an imperfect descriptor of the way human brains make decisions. Researchers have found that three distinct circuits connecting to different brain regions are involved in making good decisions, making bad ones, and determining which of those past choices to store in memory.5 Developments such as this are considered by some to raise questions about the validity of behavioural economics.6
However, the value and reliability of behavioural economics does not depend on the descriptive accuracy of dualistic decision models. It is important to remember, moreover, what Kahneman actually said about the distinction between System 1 and System 2 and how they operate. I expand on this first point below. A second point is captured by Kahneman when he writes that ‘there is no one part of the brain that either of the systems would call home’, maintaining that most mental processes involve a mixture of both systems.
Turning to the question of whether the distinction between System 1 and System 2, here meaning dualistic decision models, has a material role in practical behavioural economics, it is important to bear in mind that the discipline is highly empirical. Its applications—for example, by government bodies, by regulators of various kinds wanting to influence the decisions of consumers or firms, or by firms wanting to understand and influence the decisions of their customers7—have tended to proceed as follows.
- One identifies biases and heuristics (loosely speaking, mental shortcuts) or misconceptions (particularly of risk) where these are leading to unhelpful decisions and consequent behaviours.
- One alters the context (e.g. choice architecture) of the decisions to take account of the biases and heuristics found to be in play, and thereby improves the decisions and consequent behaviours.8
The second step can be trialled using behavioural lab experiments and/or field trials. The extent to which policy interventions based on these trials are effective depends on the experimental design and on whether the biases, heuristics and misconceptions that are being corrected are common in the affected population. Thus, efficacy does not depend on any relationship between System 1 and System 2 or on one or other of them being solely or partly responsible for the decisions of interest. The crucial underlying idea or fact is that some decisions are ‘predictably irrational’.9 What matters most in practical behavioural economics is ‘what works’.10
In line with my argument here, Andrei Shleifer, author of Inefficient Markets: An Introduction to Behavioral Finance, argues that Thinking, Fast and Slow made three separate contributions to economics.11 One was indeed the notion of System 1 and System 2. This is very important as a device for helping traditional economists to consider the consequences of decisions not always being taken by the talented and ruthless ‘rational maximiser’ embodied in homo economicus. Using Kahneman’s model, we can adduce evidence that real-world decisions are influenced by processes that might be thought to reflect System 1 and which often differ from the decisions that homo economicus would be expected to make. However, Shleifer is concerned that:12
[…] once the biology of thought is worked out, what actually happens in our heads is unlikely to neatly map into fast and slow thinking. The classification is an incredibly insightful and helpful metaphor, but it is not a biological construct or an economic model.
Shleifer proceeds to describe the two other contributions of Thinking, Fast and Slow, which are tractable in economics and therefore of critical importance in practice: heuristics and biases; and ‘Prospect Theory’, which is about the ways in which people facing decisions with associated risks fail to act in their own best interests.13
Does behavioural economics add value?
It follows from the preceding discussion that if the complexity of the human brain meant that no or few decisions are ‘predictably irrational’, then the added value of behavioural economics would be much reduced. What evidence is there for this? First of all, we may note that some behavioural treatments have much smaller effects than expected.14 This consideration, however, does not entail that there is a fundamental problem with behavioural economics. One plausible explanation is that the design of some of the remedies was suboptimal, perhaps due to the supposed multiplicity of biases. Another is that people and circumstances vary in ways that matter. I discuss other potential explanations, including bias proliferation, below.
More troubling evidence, perhaps, is the discovery that the results of some experiments in behavioural economics cannot be replicated.15 This may be due to theoretical or technical issues, or may even be the result of apparent fraud.16
Instances of fraud are likely to be highly salient when one thinks about the value and reliability of a field of science. It is important to note, however, that allegations of fraud in behavioural economics are rare and that instances of apparent fraud or serious academic misconduct occur in other areas of science without leading to a generalised claim that the relevant science is unreliable. A well-known example is the data mining carried out by the Food and Brand Lab at Cornell University when it was led by Brian Wansink.17
While I judge that there are far too few cases of proven fraud in behavioural economics for fraud itself to demand further consideration here, the extent of the replicability issue is important. Again, however, this may not reveal a fundamental problem with behavioural economics, as unappreciated fine details of situations, cultural issues or genuine data problems may be the cause of the failure to replicate. But there is, indeed, room for behavioural economics to improve, and I discuss below how this might happen. Some issues of complexity and replication must be expected to arise in behavioural economics because these are also serious problems in the underpinning field of psychology.18 These problems, though, do not undermine psychology as a whole. For example, systematic reviews of worldwide randomised controlled trials have provided strong evidence of the beneficial role of psychology in preventing self-harm.19 Thus these problems should not be thought of as undermining behavioural economics as a whole.
Under these circumstances, it is important to consider the overall track record of behavioural economics. If problems with the dualistic decision model undermined practical behavioural economics, the discipline would presumably have achieved little to date. But this is demonstrably not the case.20 For example, a wide range of nudges designed to exploit biases or heuristics in decision-making have been found to have material, beneficial effects. Stirling University’s Behavioural Science Centre has prepared a database of around 100 of these.21 For example, a field experiment in Californian shops tested the effects on purchasing behaviour of raising prices at the till or on the price tag. The result was that tax-inclusive price tags reduced demand by 8%, likely due to the salience bias, whereas being charged later at the till had no significant effect. An important recent example with widespread effects is Ofgem’s (the energy regulator for Great Britain) ‘Collective Switch Trials’. These addressed the problem that people generally would not make a very small effort to save money by switching to a preferable utilities contract (the same quality at a materially lower price). Ofgem showed that its behaviourally informed switching letters could increase the switching rate from 5% to 30%.22
The recently published book Noise by Kahneman and others can be considered to be an important update to Thinking, Fast and Slow,23 which attributed poor decisions to biases. Noise explains that some poor decisions in fact appear to be due to random noise (unexplained variability). These decisions are unpredictably irrational, which is unhelpful for economic analysis and public policymaking. Together, however, the two books point to the true complexity of decision-making discussed above, and it is this that economists, behavioural or not, must manage. As decisions based on ‘random noise’ are far more similar to decisions based on a range of behavioural biases and other psychological drivers than to the decisions of rational maximisers, Noise strengthens the case for using behavioural economics.
Equally, random noise is likely to be another reason why some well-designed behavioural trials have not had such strong results as might have been supposed. Again, however, this does not mean that behavioural economics does not or cannot add value, and as I explain above, it can and does provide useful results in practice.
Aside from ‘unexpected results’, arguably the other main doubt about behavioural economics adding value has arisen from the identification of ever more finely graded heuristics and biases. Some intimidatingly long lists of these have been prepared.24
If it were the case that each item on a long list of biases described a unique and distinct phenomenon requiring a feasible and separate treatment from other biases in the list, the list would be extremely useful, albeit very demanding to deploy empirically. There is serious concern, however, that lists of 100 or so biases lack a rigorous scientific basis—and, of course, they reduce the tractability of policy analysis and prescription. It may be the case that the incentives to come up with ‘new’ biases are unhelpfully strong. As noted by Gigerenzer:25
In [behavioural economics’] portrayal, people have systematic cognitive biases that are not only as persistent as visual illusions but also costly in real life […] such a view of human nature is tainted by a “bias bias,” the tendency to spot biases even when there are none.
Indeed, in our experience, valuable analyses can usually be done if one starts by considering whether or not one or more of just a small set of fundamental biases are likely to be present—such as reference dependence, loss aversion, mental accounting, and scarcity, salience and present biases. Empirical testing can then be used to assess whether these biases exist in the relevant context and, if so, what remedial actions can reduce them in that context. Behavioural remedies have tended to target just one bias, and it may be that their average effectiveness can be increased materially by addressing a small set of biases with different tools: individuals differ.
What of the future?
How can scientific developments in mapping the biology of the mind strengthen the practical application of behavioural economics? One way to approach this topic is to consider the current weaknesses in the practice of behavioural economics discussed above and how developments in natural science (the science of objects or processes observable in nature) could ameliorate these.
Clearly, if more were understood about how the brain makes decisions, the list of ‘biases’ for firms, governments and regulators to consider could better reflect the reality of decision-making, and could be usefully more succinct as slightly different representations of the same underlying phenomenon could be grouped together. Moreover, the role of random noise might be understood and defined in ways that enable it to be taken realistically into account. Then fewer interventions, whether commercial or for the purposes of public policy, would have unexpected results. Fortunately, some research in natural science is highly relevant to these problems and may reasonably be expected to alleviate them over time.26
One might say that, just as introducing psychology to economics through behavioural economics improved economics by making its assumptions about decision-making more realistic, natural science can in turn generate evidence to make behavioural economics more realistic. For example, the still nascent but growing field of neuroeconomics is somewhat promising.27 Here, potentially significant initial steps are now being taken. As recently as 2016, researchers reported that, ‘Despite the social and economic implications of [economic preferences for the distribution of resources], no systematic studies have examined their neural correlates’,28 which are the associations between a physical occurrence in the nervous system and a mental state or event. It now appears that one part of the brain may be associated with deliberative (selfish) processes, while another part may be associated with automatic (prosocial) processes. Much work remains to be done, but such insights open the possibility of improved direct inference of true—that is, ‘behavioural’—economic preferences, perhaps through use of brain scans as part of behavioural economics experiments. These inferences could then, for example, be included in models of behavioural industrial organisation, improving the modelling of real-world competition.
Cutting-edge behavioural economists are expected to continue to incorporate insights from other fields of study. If this happens, behavioural economics can evolve rapidly to maintain its advantage over standard economics in its areas of relevance.
Peter Andrews
1 Kahneman, D. (2011), Thinking, Fast and Slow, Farrar, Straus and Giroux.
2 For example, see Erta, K., Hunt, S., Iscenko, Z. and Brambley, W. (2013), ‘Applying behavioural economics at the Financial Conduct Authority’, Financial Conduct Authority Occasional Paper No. 1, April; Office of Fair Trading (2010), ‘What does Behavioural Economics mean for competition policy?’, OFT 1224, March.
3 Mullainathan, S. and Thaler, R.H. (2021), ‘Behavioral Economics’, The Library of Economics and Liberty.
4 Thaler, R.H. and Benartzi, S. (1994), ‘Save More Tomorrow™: Using Behavioral Economics to Increase Employee Saving’, Journal of Political Economy, 112:S1, Papers in Honor of Sherwin Rosen: A Supplement to Volume 112 (February 2004), pp. S164–S187, The University of Chicago Press.
5 Groman, S.M., Keistler, C., Keip, A.J., Hammarlund, E., DiLeone, R.J., Pittenger, C., Daeyeol, L. and Taylor, J.R. (2019), ‘Orbitofrontal Circuits Control Multiple Reinforcement-Learning Processes’, Neuron, 103:4, pp. 734–46.
6 Grayot, J.D. (2020), ‘Dual Process Theories in Behavioral Economics and Neuroeconomics: a Critical Review’, Review of Philosophy and Psychology, 11, pp. 105–36.
7 Heidhues and Kőszegi describe the interaction of rational, profit-maximising firms with customers prone to systematic errors as by far the largest topic. See Heidhues, P. and Kőszegi, B. (2018), ‘Behavioral Industrial Organization’, in B.D. Bernheim, S. DellaVigna and D. Laibson (eds), chapter 6, Handbook of Behavioural Economics, Foundations and Applications 1, North Holland.
8 UK Institute for Government (2010), ‘MINDSPACE: Influencing behaviour through public policy’, Discussion document.
9 Ariely, D. (2009), Predictably Irrational: The Hidden Forces that Shape Our Decisions, Harper.
10 See the UK government’s ‘What Works Network’, https://www.gov.uk/guidance/what-works-network.
11 Shleifer, A. (2012), ‘Psychologists at the Gate: A Review of Daniel Kahneman’s Thinking, Fast and Slow’, Journal of Economic Literature, 50:4, pp. 1–12.
12 Ibid., p. 5.
13 Shleifer describes Prospect Theory thus (ibid., p. 7): ‘Prospect Theory rests on four fundamental assumptions. First, risky choices are evaluated in terms of their gains and losses relative to a reference point, which is usually the status quo wealth. Second, individuals are loss averse, meaning extremely risk averse with respect to small bets around the reference point. Third, individuals are risk averse in the domain of gains, and risk loving in the domain of losses. And finally, in assessing lotteries, individuals convert objective probabilities into decision weights that overweight low probability events and underweight high probability ones’.
14 Smart, L. (2016), ‘Full disclosure: a round-up of FCA experimental research into giving information’, Financial Conduct Authority Occasional Paper No. 23, November.
15 See List, J. (2021), The Behavioral Economics Guide 2021, BehavioralEconomics.com.
16 Kristal, A.S., Whillans, A.V., Bazerman, M.H., Gino, F., Shu, L.L., Mazar, N. and Ariely, D. (2020), ‘Signing at the beginning versus at the end does not decrease dishonesty’, Proceedings of the National Academy of Sciences of the United States of America, 117:13, pp. 7103–7.
17 See Smith, G. (2020), ‘Torturing Data Can Destroy a Career: The Case of Brian Wansink’, 21 December.
18 Feldman-Barrett, L. (2021), ‘Psychology is in a crisis. But not the one you’re thinking of’, Science Focus, 12 August.
19 Hawton, K., Witt, K.G., Taylor Salisbury, T.L., Arensman, E., Gunnell, D., Hazell, P., Townsend, E. and van Heeringen, K. (2016), ‘Psychosocial interventions for self-harm in adults’, Cochrane Database of Systematic Reviews, 12:5, doi:10.1002/14651858.
20 Rutter, J. (2014), ‘MINDSPACE grows up – behavioural economics in government’, UK Institute for Government, 6 January.
21 Egan, M. (2020), ‘Nudge Database v1.2’, University of Stirling.
22 Ofgem (2019), ‘Ofgem’s Collective Switch Trials’, Report, 27 September.
23 Kahneman, D., Sibony, O. and Sunstein, C.R. (2021), Noise: A Flaw in Human Judgment, Collins.
24 See, for instance, the extensive list of cognitive biases on Wikipedia: https://en.wikipedia.org/wiki/List_of_cognitive_biases.
25 Gigerenzer, G. (2018), ‘The Bias Bias in Behavioral Economics’, Review of Behavioral Economics, 5, pp. 303–36.
26 Murray, E. and Wise, P. (2010), ‘Interactions between orbital prefrontal cortex and amygdala: advanced cognition, learned responses and instinctive behaviors’, Current Opinion in Neurobiology, 20:2, pp. 212–20.
27 Konovalov, A. and Krajbich, I. (2019), ‘Over a Decade of Neuroeconomics: What Have We Learned?’, Organizational Research Methods, 22:1.
28 Fermin, A.S.R., Sakagami, M., Kiyonari, T., Li, Y., Matsumoto, Y. and Yamagishi, T. (2016), ‘Representation of economic preferences in the structure and function of the amygdala and prefrontal cortex’, Scientific Reports, 6, Article number: 20982.
Related
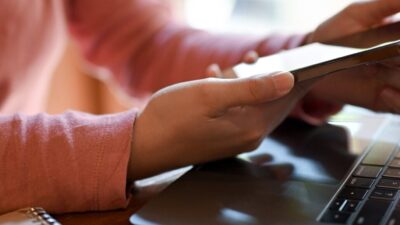
Economics of the Data Act: part 1
As electronic sensors, processing power and storage have become cheaper, a growing number of connected IoT (internet of things) devices are collecting and processing data in our homes and businesses. The purpose of the EU’s Data Act is to define the rights to access and use data generated by… Read More
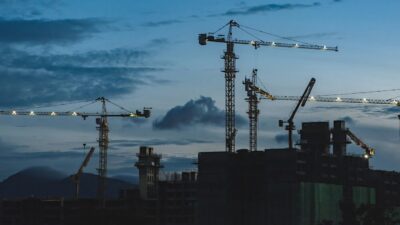
Adding value with a portfolio approach to funding reduction
Budgets for capital projects are coming under pressure as funding is not being maintained in real price terms. The response from portfolio managers has been to cancel or postpone future projects or slow the pace of ongoing projects. If this is undertaken on an individual project level, it could lead… Read More