When algorithms set prices: winners and losers
The digital revolution has led to a significant growth in companies’ ability to capture, store and analyse data about their customers, competitors and the wider world. Increasingly, companies are using this information to develop algorithms that set prices for them. But how might the automation of pricing through algorithms affect competitive outcomes in markets, and result in different consumers being charged different amounts for the same good or service?
This article is based on Oxera (2017), ‘When algorithms set prices: winners and losers’, discussion paper, 19 June. The discussion paper was inspired by a meeting in May 2017 of the Oxera Economics Council, a group of leading European academics and officials of the European Commission. This article does not reflect the views of the Council or its members.
The risk that price-setting algorithms using artificial intelligence (AI) could collude among themselves, to the detriment of consumers, has received significant media attention. Academics, Ariel Ezrachi and Maurice Stucke, were among the first to point out this risk,1 and their work has influenced several speeches and comments by representatives of competition authorities and global organisations, including the European Commissioner for Competition, Margrethe Vestager,2 and the Organisation for Economic Co-operation and Development (OECD).3
At the same time, others suggest that the use of algorithms can be efficient and procompetitive, leading to outcomes that benefit consumers through faster adjustments to prevailing market conditions.
This article explores these two contrasting positions. While the impact of algorithms that use simple rules or formulae to set prices can be assessed in a relatively straightforward way, it is more difficult to judge the more advanced algorithms. These increasingly use AI to adapt and learn as they experience new situations. The way in which AI-driven algorithms learn is highly complex, and, typically, it is not possible to determine why they result in a particular outcome. An outsider cannot ‘reverse engineer’ the algorithm.
Why do companies use algorithmic pricing?
Algorithmic pricing has clear efficiency advantages for companies that use it—it can be cost-reducing, revenue-increasing, or both.
In some cases, this form of pricing is central to the existence of the market in the first place: for example, it is hard to imagine how the online advertising market could function at anything close to the scale it does without automated pricing procedures—in this case, based mostly around automated online auctions. This matters for consumers, as online advertising is the source that enables many online services to be offered free of charge to consumers.
Algorithmic pricing is likely to occur in markets where:
- the costs to serve consumers differ considerably from consumer to consumer, in ways which can be approximated using observable data (e.g. credit and insurance markets);
- demand fluctuates much more rapidly than supply (e.g. hotels and ride-sharing);
- the price-setter has a wide range of products to price, and algorithmic approaches bring a significant cost advantage (e.g. consumer retail).
Companies’ motivations for using algorithmic pricing in each of these areas are clear. But how does it influence competition, and therefore outcomes for consumers? And are there winners and losers?
How does algorithmic pricing affect competition and consumers?
While many commentators have focused on the likely problems with algorithmic pricing, its many positive features have the potential to enhance outcomes for consumers. This is partly due to how algorithms ‘learn’ from previous experience, as shown in the box.
Reinforcement learning framework
An algorithm might simply measure responses to previous changes in supply or price, in order to predict what would happen in similar situations in future. This information can then be used to adjust prices to deliver a desired outcome.
More advanced algorithms may employ a ‘reinforcement learning’ approach to real-world data to continuously learn how to set prices, as depicted in the figure. These advanced algorithms take into account the link between actions (e.g. setting prices) and states (e.g. stock levels and competitors’ prices), and how actions today can affect future rewards (e.g. how competitors might respond to price changes and the stock available to be sold in future periods). The most sophisticated approaches also allow for the possibility that the relationships will change over time.
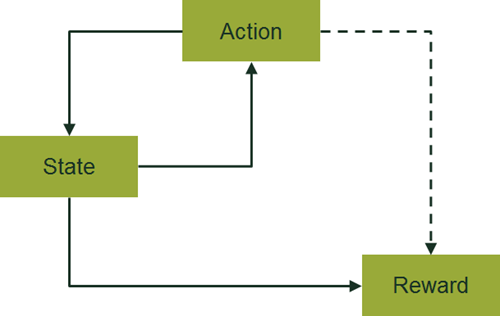
Source: Oxera.
Algorithms allow for faster and more accurate price adjustments, taking into account extensive market information. This should improve the matching of fluctuating demand and supply, which makes markets work more effectively and can result in better outcomes for consumers in the form of lower prices or their demands being better met—for example, through shorter waiting times for a ride during peak times. Algorithms can also substantially reduce the costs of setting and changing prices, and facilitate entry by new suppliers, as they can quickly learn how a market works. The example of Airbnb is described in the box below.
Airbnb: pricing a diverse range of products
Airbnb is a marketplace that connects guests with local hosts seeking to rent out available space. Hosts can set prices freely, but Airbnb recommends prices to them according to an algorithm that incorporates machine learning. The price recommendations are based on criteria such as location and size, the property’s occupancy rate, booking duration, season, and competitors’ prices and availability. The price tips vary over time—for example, to take into account local events—and are updated regularly.1
As most hosts do not engage in letting as their primary business,2 the recommendations can help them to quickly and effortlessly find the ‘right’ price for their accommodation, as an alternative to trial and error. On the downside, Airbnb might have an incentive to recommend higher prices than the hosts would set themselves (in order to maximise commissions), and thereby raise the overall price level on the platform.
Note: 1 See Yee, H. and Ifrach, B. (2015), ‘Aerosolve: Machine learning for humans’, Airbnb, 4 June, accessed 21 June 2017. 2 Airbnb (2017), ‘New Study: Airbnb Community Generates £502 Million in Economic Activity in the UK’, accessed 21 June 2017.
Algorithmic pricing can also intensify competition directly. By speeding up responses between competing suppliers, prices may converge to competitive outcomes more rapidly than they would do otherwise.
On the downside, some approaches to algorithmic pricing may be better at sustaining tacitly coordinated outcomes than when prices are set by humans. In particular, algorithms have increased capacity to monitor price movements in the market, and are faster at reacting to changes. In theory, this could enable algorithms to reach collusive outcomes more frequently. In certain situations, algorithms can independently learn to avoid price wars, as a way of maximising profits over the longer term. This could harm consumers, who would not see the lower prices that competition can deliver. The degree to which such collusion among algorithms is likely to happen in practice is not yet clear.
Algorithms may also be able to facilitate vertical agreements (i.e. those between firms in a supply chain) or collusion through a common vertical agent in the market. For example, if many companies use algorithm software from the same provider, one firm’s algorithm could anticipate the reaction of those of others, and hence be able to set higher prices. Similar concerns might apply to a platform such as eBay or Amazon Marketplace, through which companies sell their products, and which is involved in setting prices.
Thus, algorithmic pricing may require new approaches to competition investigations, and possibly even to the legal definition of competition infringements. Algorithms that reach tacitly coordinated outcomes will, by their nature, be difficult to identify and interpret. Competition authorities will need to think not only about the tools used to identify issues, but also what constitutes an illegal act when algorithms interact. Likewise, companies using algorithms will need to review and test their pricing practices from a legal and economic perspective to avoid infringing competition law.
While much of the debate in relation to algorithms has been around competition law, there are also important distributional implications of algorithmic pricing—i.e. different consumers paying different prices for the same product. Regulators in sectors such as financial services and telecoms are increasingly looking into these issues.
What are the distributional implications of algorithmic pricing?
One feature of the digital economy is that, despite the availability of a large amount of data on consumers’ characteristics, attitudes and preferences, there is as yet relatively little evidence of widespread personalised pricing, other than in industries that have always relied on customer-specific pricing, such as insurance. Many subscription services (e.g. for music streaming and on-demand video) have flat rates across all (or groups of) customers, even though usage, while predictable, varies considerably across consumers, as do the associated costs.
It has been shown that consumers do not like personalised prices. Early experiments by Amazon in setting variable prices for individuals4 saw an overwhelmingly negative response, even if some consumers probably paid less as a result. This ‘punishment’ of companies by consumers for treating them differently may be a good reason why we have not seen mass adoption of personalised pricing across digital markets.
What algorithmic pricing is doing, however, is disrupting large swathes of cross-subsidisation in many markets—especially where the costs to serve different customers can vary significantly, such as in the credit and insurance sectors.
Therefore, while setting uniform prices for all consumers may seem fair, it could be hiding cross-subsidisation between different groups of consumers, and this cross-subsidisation itself may not always be considered fair. For example, it is cheaper for banks to serve consumers who use Internet banking than those who visit their branches, and yet often all consumers face the same charges for their current account, or receive the same interest rate.
While cross-subsidisation can be economically inefficient, it can also protect consumers. For example, regulators are often concerned about the prices paid by vulnerable consumers relative to those paid by ‘sophisticated’ consumers. Preventing price discrimination can be a way to ensure that competition for sophisticated consumers benefits vulnerable consumers as well.
Price discrimination is driven not only by the cost to serve customers, but also by customers’ willingness to pay or to switch provider. Algorithmic approaches to pricing may identify and exploit these differences between consumers more effectively than prices set by humans.
In this context, what constitutes a fair price? Fairness is hard to define economically, but notions of fairness do exist. In particular, there is an increasing amount of media attention and academic research on algorithms that are used to assess reoffending risk in the US criminal justice system, and whether these algorithms produce results that are ‘fair’.5 Many of these ideas translate directly into scenarios where some measure of risk is relevant to cost, such as in the credit or insurance sectors.
The example of insurance, and the regulatory response to risk-based pricing in the sector, is given in the box below.
Insurance products: compensating for risks
In the market for insurance products, such as motor and home insurance, costs are typically driven by the level of risk of the policyholder (e.g. driven by past claims or location) and that of the insured item (e.g. car type). Risk-based pricing can make insurance markets work more effectively by reducing cross-subsidisation between consumers.1 In practice, it means that insurance products are individually priced.
From using algorithms to set prices for insurance products according to risk characteristics, it is a small step to using them in assessing willingness to pay. Insurance providers gather an extensive amount of data about customers. For example, employment status and car type can provide clues about willingness to pay and whether someone is likely to shop around. In practice, providers can then offer lower prices to attract new customers (switchers) and steadily increase them for those who remain with the insurer over time.
However, the use of algorithmic pricing in insurance markets has also raised policy and fairness questions in situations where factors that predict the level of risk are correlated with consumer characteristics such as ethnicity, age and gender. Pricing along these dimensions is illegal, discriminatory or considered unfair. However, it is not clear whether regulating the market by banning the use of certain inputs will remain effective going forward, as algorithms become more sophisticated and amass a greater level of detailed consumer data. Algorithms might be able to use alternative information to predict ‘banned’ consumer characteristics. For example, the EU ban on the use of gender in car insurance pricing resulted in a reliance on other information to infer the gender of the applicant—for example, male drivers typically drive bigger cars or are more likely to work in certain industries. The ban ultimately may have led to a widening of the gap in insurance premiums.2
Note: 1 For a discussion, see Oxera (2010), ‘The use of gender in insurance pricing: unfair discrimination?’, Agenda, September. 2 See Collinson, P. (2017), ‘How an EU gender equality ruling widened inequality’, The Guardian, January.
Are algorithms good for us?
Algorithms are opening up whole new markets, allowing new entrants to operate in existing markets, and helping some consumers get better value for money. But they pose new challenges to policymakers, regulators and competition authorities. Traditional approaches to spotting collusive activity, for example by incentivising whistle-blowers, are less likely to work with algorithms. Also, it is unclear what constitutes evidence of collusive activity in an environment where there is no record of pricing decisions, and where algorithms are making autonomous decisions based on public-domain information.
It is important to note that the two broad concerns about algorithmic pricing are unlikely to arise simultaneously in any specific market. Markets with characteristics that may make them amenable to collusion tend to be less favourable to personalised pricing. Markets where personalised pricing is prevalent do not easily lend themselves to collusion.
The framework for enforcing competition law needs to adapt to a world of algorithmic pricing. This could include monitoring digital markets in much more automated ways, building test environments where the algorithms of companies under investigation can be examined to see how they react to shocks, or asking companies to consider the distributional effects of their pricing policies.
Algorithms are here to stay. Competition authorities and regulators will need to adapt to this new world. Yet it is also important to keep in mind that algorithms can help to break down barriers to competition and make markets more effective.
1 Ezrachi, A. and Stucke, M.E. (2016), Virtual Competition: The Promise and Perils of the Algorithm-Driven Economy, Harvard University Press.
2 Vestager, M. (2017), ‘Algorithms and competition’, speech, Bundeskartellamt 18th Conference on Competition, Berlin, 16 March.
3 OECD (2017), ‘Algorithms and Collusion – Background Note by the Secretariat’, 9 June, DAF/COMP(2017).
4 Streitfeld, D. (2000), ‘On the Web, Price Tags Blur’, The Washington Post, 27 September.
5 See Lum, K. and Isaac, W. (2016), ‘To predict and serve?’, Significance, 13:5, October, pp. 14–19; and Larson, J., Mattu, S., Kirchner, L. and Angwin, J. (2016), ‘How We Analyzed the COMPAS Recidivism Algorithm’, ProPublica, 23 May.
Download
Related
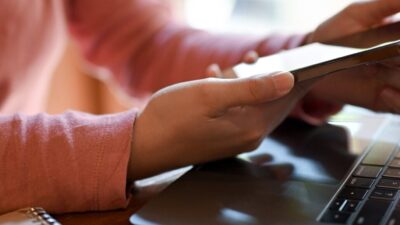
Economics of the Data Act: part 1
As electronic sensors, processing power and storage have become cheaper, a growing number of connected IoT (internet of things) devices are collecting and processing data in our homes and businesses. The purpose of the EU’s Data Act is to define the rights to access and use data generated by… Read More
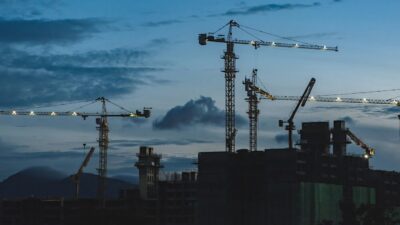
Adding value with a portfolio approach to funding reduction
Budgets for capital projects are coming under pressure as funding is not being maintained in real price terms. The response from portfolio managers has been to cancel or postpone future projects or slow the pace of ongoing projects. If this is undertaken on an individual project level, it could lead… Read More