Setting the efficiency for NHS Trusts: has Monitor got the balance right?
The 2015/16 budget for the NHS in England is £116.4bn—equivalent to around 7% of UK gross domestic product (GDP). Faced with a combination of rising costs, increasing patient demand and a UK government commitment to run a budget surplus in ‘normal’ times, NHS funding has come under increasing pressure in recent years. Consequently, incentivising and delivering efficiency improvements have become key priorities for the NHS.
Under the Health and Social Care Act 2012, Monitor1 is responsible for setting national tariffs on an annual basis for a range of healthcare services—for example, services involved in providing care to patients admitted to hospital, outpatient care, and emergency care. To drive value for money, Monitor seeks to set prices that reflect efficient costs, and incentivises NHS Trusts to reduce costs over time by finding more efficient ways of working.2 This needs to be balanced against the need for the Trusts to maintain and improve their services in terms of safety, quality, level of integration, and access.
For 2016/17, Monitor has set national tariffs based on rolled-over prices from the previous year.3 A sector-wide efficiency factor of 2% is proposed to be overlaid on this.4
This article reviews the methodology underpinning Monitor’s analysis in deriving the efficiency factor,5 contrasting it with approaches and frameworks considered by utility regulators in the UK, and makes some suggestions for the development and application of Monitor’s analysis.
Contrasting Monitor with UK utility regulators
In a simple textbook world, healthcare providers in England would be free to set prices. Health market dynamics would be such that providers would have incentives to deliver services efficiently and effectively. Patients would identify which providers offer the best quality of care, and at the lowest price, and it is these providers who would gain at the expense of those providing poorer value for money.
However, healthcare in England does not work like this. First, most NHS care is free at the point of delivery. As such, patients cannot know whether the services offer value for money. Second, while patients may exercise some choice over elective care, they have much less choice in relation to emergency care. Given these factors, many NHS treatments are subject to a national tariff, against which providers are remunerated.
The combination of choice (exercised by elective patients seeking higher-quality treatment), and administrative action by the various regulators in health and social care,6 is designed to produce a system whereby NHS institutions are presented with an incentive to improve their efficiency of operation. This then feeds into the tariff-setting process, which mirrors the RPI – X approach adopted by the regulated monopoly utilities.
Undertaking comparisons across peers and setting efficiency targets is common practice among utility regulators in the UK as a means of replicating some of the outcomes of a competitive market. In particular, it can create an incentive for the providers to seek out and implement efficiency gains while also passing those gains on to consumers sooner than might otherwise be the case.
In this context, utility regulators often use cost benchmarking to set cost- or price-reduction targets for organisations to achieve during the (typically multi-year) price control period, or as a basis against which to monitor the organisation’s performance. Many organisations also undertake benchmarking to determine their own internal efficiency challenges for business planning purposes (or in presenting well-justified business plans to the regulator).
In the box below, we compare the economic framework commonly considered by utility regulators to determine the efficiency factor with the approach adopted by Monitor.
Monitor’s approach to deriving the efficiency factor for 2016/17 involves comparing cost performance at the organisation level (in this case, NHS Trusts) and over time using econometric modelling. Its use of a panel data7 framework is in line with the majority of the UK utility regulators’ approaches. Ofwat (water),8 Ofgem (energy),9 and the Office of Rail and Road (ORR)10 have all relied heavily on panel data modelling techniques in their most recent price control reviews.
In Monitor’s case, modelling at the overall Trust (i.e. aggregate) level is a pragmatic decision. This is because the data from which the efficiency factor is derived could be subject to significant error or short-term volatility at a more disaggregated level, at least with the currently available data.11 In a utility regulation context, once a mechanism for collecting comparable and consistent data is established, modelling at different levels of aggregation is typically considered12 because aggregated and disaggregated modelling have their advantages and disadvantages.13
In terms of benchmarking approaches, Monitor has used a panel data stochastic frontier analysis (SFA)14 model and a random effects (RE) model.15 Both of these have been used in a regulatory context in the UK and Europe. For example, the ORR16 and Ofcom17 have considered a panel SFA approach as part of the cost assessment toolkit. In Europe, SFA (alongside data envelopment analysis, DEA) appear to be the commonly used techniques.18 Similarly, the ORR, Ofwat and Ofgem have considered a RE model in the most recent price control reviews.19
Finally, in contrast to the majority of other utility regulators, Monitor has incorporated service quality levels (e.g. quality of care based on patient satisfaction surveys) within cost assessment directly. Regulators such as Ofwat20 and Ofgem21 have recently indicated that they are seeking to integrate customer outcomes and quality of service within their cost benchmarking framework.
In summary, while the economic framework considered by Monitor differs slightly from utility regulators, the benchmarking approaches employed are broadly in line with regulatory precedents. In addition, Monitor integrates service quality levels within cost assessment, a development that other regulators are seeking to implement in future price reviews.
Reviewing Monitor’s efficiency methodology
Here, we briefly discuss some areas where Monitor’s methodology could be developed further, or where the application of the efficiency factor may need careful consideration.22
- A uniform efficiency factor may not be appropriate for all Trusts. Monitor’s 2% efficiency factor (based on a combination of catch-up and frontier-shift efficiencies) applies to all Trusts. However, this may not be appropriate for some of the Trusts. For example, for those in the middle of the efficiency spectrum, which have historically improved their efficiency by catching up to best practice, using historical performance to inform the target could be challenging since some of the historically achieved efficiency gains may not be replicable.23
- The implicit assumption that Trusts have not converged in performance may lead to upward bias in the efficiency factor. Monitor’s efficiency analysis seeks to determine the average efficiency improvements achieved by the Trusts over the historical period and applies this to the sector-average efficient prices. Monitor’s econometric models assume that there is no convergence in the performance of the relatively inefficient Trusts over the period of analysis. While Monitor does mention some evidence on the lack of catch-up,24 this assumption does not appear to be empirically tested on the data used to determine the average rate of improvement. This could result in potential upward bias in the measured efficiency factor, and the projected efficiency factor could be unachievable as the potential for further catch-up will be diminished. The box below illustrates this point further.
- There is relatively limited cross-checking of results with other efficiency approaches. The modelling approach adopted by Monitor is econometric and top-down in nature, which has its limitations.25 To ensure that the assumptions imposed on the model are not driving the result, it would be useful to cross-check the results from models using alternative assumptions, and from other modelling approaches. For example, to determine the energy networks’ relative efficiencies in the RIIO price controls, Ofgem used a toolkit of approaches (including econometric benchmarking at different levels of aggregation and bottom-up assessments). In this way, the results from the different approaches can be compared and contrasted, and, based on an understanding of the approaches, some consensus could be reached to identify a robust range for the estimated inefficiencies.
On this last point, in utility regulation, according to surveys, SFA and DEA seem to rank as the most commonly used approaches.26 In Monitor’s case, DEA could be particularly useful as it can readily provide measures of frontier shift (i.e. trend efficiency) that is distinct from Trust-specific efficiency change over time (i.e. variation in efficiency).27 DEA could also allow Trusts to readily identify their ‘peers’ and facilitate sharing of best practice. Similarly, more disaggregated, bottom-up or operational evidence is often used as a cross-check in a regulatory context.
What happens next?
The tariff-setting approach for the NHS is an evolving process, as is the case in network utilities. Getting the ‘right’ efficiency factor involves ensuring that the economic framework, model development process, and efficiency approach underlying the efficiency factor are robust, transparent, and consistent with economic best practice.
Monitor, in setting efficiency targets in its overall regulatory structure, has to strike a careful balance between incentivising quality healthcare and driving down costs. It is conscious that a non-robust application of efficiency analysis may result in unachievable targets.28 In particular, the management of NHS Trusts need to be given realistic objectives in relation to the evolution of their costs, which will be funded via the future tariffs that Monitor sets. If future prices are set too high, difficult management decisions necessary to improve efficiency may not happen soon enough. If tariffs are set too low, management may become demotivated, as they may not be able to meet the efficiency targets set, whatever they do, with implications for financial sustainability.
In terms of the overall direction and intention of the modelling undertaken by Monitor, there are many merits to its approach in setting the efficiency factor. This article has identified some potential areas for the development and application of Monitor’s analysis that it may wish to consider when determining the efficiency factor for 2017/18. Further improvements may also be possible in undertaking future assessments. The suggestions set out above could result in a more robust estimation of current and potential levels of efficiency, both across Trusts and over time. This could help secure the right balance between reducing unit costs and maintaining quality care for patients.
Contact: Srini Parthasarathy
Download
Related
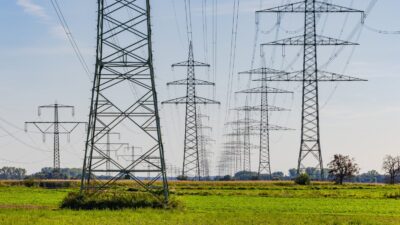
Ofgem’s RIIO-3 Sector Specific Methodology Decision
On 18 July 2024, Ofgem published its Sector Specific Methodology Decision (SSMD) for the forthcoming RIIO-3 price control period for electricity transmission (ET), gas transmission (GT) and gas distribution (GD) networks.1 This follows Ofgem’s consultation on the matter in December 2023.2 RIIO-3 will last for… Read More
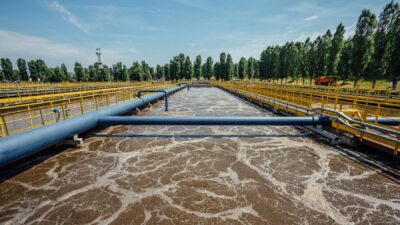
The future funding of the England & Wales water sector: Ofwat’s draft determinations
On Thursday 11 July, Ofwat (the England and Wales water regulator) published its much anticipated Draft Determinations (DDs). As part of the PR24 price review, this sets out its provisional assessment of allowed revenues and performance targets for AMP8 (2025–30)—and will be of great interest to water companies, investors,… Read More