Causality and natural experiments: the 2021 Nobel Prize in Economic Sciences
The Royal Swedish Academy of Sciences awarded the Sveriges Riksbank Prize in Economic Sciences in Memory of Alfred Nobel 2021 to three economists—Joshua Angrist, David Card, and Guido Imbens. Their contributions to the economics literature shaped economists’ understanding of when causal relationships can be established, especially using non-experimental data, and what kinds of methods and assumptions allow us to uncover the true causal effect of one variable on another. Today, businesses, courts and policymakers rely on causal empirical evidence to make their decisions.
The Sveriges Riksbank Prize in Economic Sciences in Memory of Alfred Nobel 2021 is shared by three economists.
- David Card received half of the prize ‘for his empirical contributions to labour economics’.
- Joshua D. Angrist and Guido W. Imbens shared the other half of the prize ‘for their methodological contributions to the analysis of causal relationships’.1
Alan Krueger
Another prominent economist made a great contribution to the literature and research agenda on causal inference alongside the 2021 Nobel Prize winners. We have no doubt that Alan Krueger would have shared this award—however, he passed away in 2019, and Nobel Prizes are not awarded posthumously.
The common theme for the 2021 Nobel Prize is causality and natural experiments.
Why causality matters
Many policy and business decisions require a thorough understanding of causes and effects. They might involve questions such as:
- will a higher minimum wage cause unemployment?
- how much will a person’s income increase if they have one more year of schooling?
- by how much will a company’s sales decrease if it increases its prices?
- what is the damage resulting from a particular cartel agreement?
However, naïve approaches to analysing data in order to answer such questions may result in policy recommendations, or decisions, that are based on a misunderstanding of the effects of a factor on an outcome of interest.
Answering such questions requires an approach that goes beyond using data to explore mere correlations—since relationships observed in data are not necessarily informative of causal effects if they are not collected and analysed using the right approaches. The field of empirical economics is devoted to understanding these approaches, especially in cases where it is difficult to follow the ‘gold standard’.
The gold standard in identifying empirical causal relationships is arguably randomised control trials (RCTs). For example, in medicine it is common practice to randomly allocate a medical treatment to some participants in a medical trial and, in parallel, define a comparable control group of non-treated participants who usually receive a placebo treatment. Outcomes for the treated and non-treated participants are then compared to identify the effectiveness of the treatment. Such trials were undertaken in 2020 and 2021 in order to test the efficacy of COVID-19 vaccines. The key factor that means that such trials can identify the causal impact of a treatment on patient health is the randomisation between who receives the treatment and who does not.
For example, Figure 1 gives a simplified representation of the relationship between the height and intelligence of hypothetical individuals in a hypothetical society, and illustrates how it varies depending on the sample selected.
Figure 1 Non-random samples can severely mischaracterise underlying relationships (sampling bias)
Note: Please use the selector to display a particular sample. The option ‘All society’ displays all data points. ‘Non-random selection’ displays a particular sample that is not selected randomly. ‘Random selection’ displays observations that are selected randomly from the underlying society. Lines show the line of best fit to the displayed sample. The figure illustrates that the linear relationship estimated using the non-random selection is significantly different from the relationship in the whole society, whereas the relationship estimated using the random selection closely approximates it.
Source: Oxera.
Common theme: natural experiments
A natural experiment can be considered an approximation of a RCT—when it is impossible or unethical to randomise a treatment across participants. Natural experiments arise when nature, or policies, result in situations where a treatment is automatically assigned to participants in a manner that is ‘almost as good as random’. The term ‘treatment’ is thereby not limited to medical treatment. It covers anything that might have an effect on an outcome—for instance, education on earnings, gender on pay, or a cartel on prices.
Treatments that are used to answer many empirical questions in economics would be impossible to randomise across participants. For example, among many other social issues, Angrist and Krueger analysed the impact of educational attainment on workplace earnings.2 To study this empirical relationship, and to obtain a dataset with a randomised treatment, the authors would have had to assign different years of schooling to different children randomly and compare their future earnings. Such a research design is unethical, and an RCT is therefore not a suitable research method to answer this question.
Angrist and Krueger instead used a natural experiment arising from US legislation—the compulsory education leaving age. They argued that this legislation results in a natural experiment, as students who are born earlier in the calendar year are slightly older, and therefore reach the compulsory education leaving age earlier than their peers (born later in the calendar year). As such, people born earlier in the calendar year tend to have less education than those born later even though the birth date of an individual is random. The legislation therefore arguably results in an ‘almost as good as random’ allocation of years of schooling.
Card and Krueger showed another example of how natural experiments can be used to identify causal effects—in their case, to understand the impact of a minimum wage on employment in the USA, and specifically whether raising the minimum wage costs jobs.3 They used data arising from a policy change4 along with a suitable analytical approach.5 Specifically, the authors compared the evolution of employment metrics in New Jersey, a state with a change in its minimum wage rate policy, and the evolution of the same employment metrics in neighbouring Pennsylvania, a state without the change in minimum wage. In this case, ‘assignment’ of the treatment can be considered ‘almost as good as random’ across New Jersey and Pennsylvania. The authors found ‘no indication’ of a negative impact on jobs in fast-food chain restaurants in the two states (shown in Figure 2 below). Such innovative use of data has influenced generations of empirical economists who have used natural experiments.
Figure 2 Geographical boundaries are sometimes used to differentiate policy impacts across similar units
Note: The bright green line represents the border between Pennsylvania and New Jersey. Light and dark green circles represent some of the fast-food restaurants near the border. Fast-food restaurants are selected randomly for illustration only and are not necessarily part of Card and Krueger’s data. In 1992, restaurants on the Pennsylvania (left-hand) side of the border received the treatment (increased minimum wage), and those on the New Jersey (right-hand) side of the border did not. Considering how close these groups of restaurants are to each other, it might be reasonable to assume that the group of New Jersey restaurants (dark green circles) represent a reasonable control group to measure the impact of a treatment on the group of Pennsylvania restaurants (light green circles).
Source: Oxera.
A fundamental difference between natural experiments and RCTs is the researcher’s ability to control who receives the treatment. In an RCT, a researcher allocates participants randomly to treatment and control groups without giving them a choice. However, as natural experiments are by their nature uncontrolled, there can be various situations where individual responses to treatment vary. When such situations arise, the interpretation of the differences in outcomes becomes challenging.
For example, in the Angrist and Krueger study on the impact of educational attainment on earnings, one could look at who the legislation on compulsory schooling really affects. We are asked to consider compulsory schooling as an intervention affecting all students; however, it actually affects the behaviour only of those who would have left education absent the legislation on compulsory schooling—other students would have completed their education anyway. The impact of the compulsory schooling treatment varies across participants.
Imbens and Angrist developed the analytical framework to use in such situations, which shaped how researchers use, and think about, natural experiments.6 They showed that what can be identified under certain assumptions is the impact of treatment only on those whose behaviour is altered as a result of the treatment, and name this outcome the ‘local average treatment effect’.
How does regression analysis fit alongside these concepts?
RCTs and natural experiments have the advantage that their random or almost random assignment does much of the work to uncover the effect, and the statistical analysis itself can be simple. However, RCTs and natural experiments (as with neighbouring states subject to different policy changes) are not always available to researchers or informative about the underlying mechanisms. Nonetheless, the idea behind them both—in other words, how randomisation and causal inference are linked—affects how causal research is done by today’s organisations.
If these approaches are unavailable, other statistical approaches can be used instead. Notably, without the benefits of randomisation, regression approaches start by defining a causal model of assumed relationships, therefore giving an explicit assumed structure to how one factor affects another. When these assumed relationships are correct, regression outcomes are informative about the causal effect and the mechanisms through which it takes place.7 To ensure adherence to the ‘almost as good as random’ principle, regression approaches need to ‘control’ for the effects of not just the principal variable of interest but all relevant factors (the ‘confounders’).8 If these can all be accounted for, a regression approach allows the researcher to identify and measure the effect of the main variable of interest.
A practical example might be a cartel case where we might expect prices to increase due to the formation of the cartel. However, prices depend on many factors, of which the cartel conduct is only one. Such an analysis might rely on a comparison of prices during the cartel period with prices after it. As prices might also change over time due to (for instance), input costs, demand, or product characteristics, simply comparing averages between two points in time would not measure the cartel effect but all the effects together, and would be uninformative about individual components. Only if all other relevant factors are accounted for, and the modelling assumptions hold, is it is possible to measure the actual effect of the cartel on prices.
Figure 3 shows observations on price and demand in two hypothetical markets.
Figure 3 Regression approaches control for confounders to assess the relationship
Note: The relationship between prices and demand is simplified for illustrative purposes. If differences in market characteristics are not accounted for, a regression of demand on prices results in a counterintuitive positive relationship. Only after controlling for market characteristics does a regression approach help to uncover the expected negative relationship. The animation shows a step-by-step guide to how this is done.
Source: Oxera.
Natural experiments and causal research in today’s applied economics
An understanding of cause-and-effect relationships is not only relevant in the public policy space but also plays an increasingly important role in business decision-making. For example, companies may want to know how much more profit they can generate from a better customer experience specifically, separately from any impacts of changes in product quality or differences in customer demographics.
In this example, a company could choose the ‘controlled experiment’ route—i.e. running an experiment in one region of the market and comparing changes in its profits against changes in a similar region that does not go through the treatment. Alternatively, if an experiment is not feasible, it could run a regression analysis examining the impact of different levels of customer experience on profits, controlling for all other relevant factors. In both approaches, the experimental design and implementation, as well as the analysis of the impact, would require careful consideration.
Indeed, a growing number of companies are applying economic analysis to understand causal relationships and the impacts of their business decisions in many areas—such as product design, marketing, pricing, and arrangements with suppliers/buyers. Tech companies are perhaps at the forefront of applying economic analysis in the design of platforms, from more high-level questions on sustainable business models to more detailed ones on platform features and the customer journey.9
Oxera uses natural experiments as part of its analytical toolkit. For example, in the context of a recent merger assessment, our team assessed the closeness of competition between two airlines that intended to merge. If the parties were close competitors, the merger might have led to a lessening of competition and an increase in prices. As part of the assessment, the team undertook a case study that looked at how prices evolved on a route following the grounding of Boeing 737 MAX aircraft due to safety concerns. One of the parties to the merger (airline A) operated 737 MAX aircraft on the route—and was therefore affected by this grounding event—while the other (airline B) did not. This situation provided a natural experiment that the team used for its analysis. Because this negative supply shock (the grounding of one type of aircraft) yielded an unexpected output decline for airline A, it provided useful information about the competitive reactions of other airlines flying on that same route. Oxera’s analysis indicated that the parties impose material competitive constraints on each other: the grounding event resulted in airline B increasing its prices on the route, and when airline A started operating the route using different aircraft, airline B’s prices subsequently fell.
Looking to the future
Generations of researchers have been inspired by the approaches that the 2021 Nobel winners have pioneered. As practitioners of applied economics, we are looking forward to their future contributions to this field, which may expand the economist’s analytical toolkit with various data science approaches.
For example, Imbens has recently worked on understanding how prediction-focused data science approaches can be translated to causal settings and when they can be useful.10 It is exciting to see developments in these areas as they illustrate the growing ability of economic research designs to capture true underlying effects, which will result in better and more accurate data-driven policy and business decisions in the future.
1 The Nobel Prize (2021), ‘Press release: The Prize in Economic Sciences 2021’.
2 Angrist, J.D. and Krueger, A.B. (1991), ‘Does compulsory school attendance affect schooling and earnings?’, The Quarterly Journal of Economics, 106:4, November, pp. 979–1014.
3 Card, D. and Kruger, A.B. (1994), ‘Minimum Wages and Employment: A Case Study of the Fast Food Industry in New Jersey and Pennsylvania’, The American Economic Review, 84:4, September, pp. 772–93.
4 On 1 April 1992, the minimum wage rate in New Jersey was increased from $4.25 to $5.05 per hour.
5 They compared employment, and other labour metrics such as wage, between New Jersey and its neighbouring state Pennsylvania before and after the policy change. This analytical approach is more widely known as a ‘difference-in-differences’ approach.
6 Imbens, G.W. and Angrist, J.D. (1994), ‘Identification and estimation of local average treatment effects’, Econometrics, 62:2, March, pp. 467–75.
7 This comes at the cost of various modelling assumptions that are not required when using RCTs or natural experiments.
8 Technically, this means that once all common causes (confounders) of an outcome and treatment assignment mechanism are included in the regression in suitable forms—i.e. once the other effects are controlled for—the assignment to one group or the other is ‘almost as good as random’. Controlling for common causes allows researchers to mimic random assignment when the modelling assumptions hold.
9 For example, see the work by Amazon’s Core AI group: Bajari, P., Cen, Z., Chernozhukov, V., Huerta, R., Li, J., Manukonda, M. and Monokroussos, G. (2020), ‘New Goods, Productivity and the Measurement of Inflation: Using Machine Learning to Improve Quality Adjustments’, American Economic Association, January.
10 See, for instance, Athey, S. and Imbens, G.W. (2019), ‘Machine learning methods that economists should know about’, Annual Review of Economics, 11, August, pp. 685–725.
Related
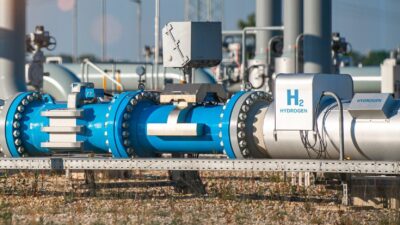
Time to get real about hydrogen (and the regulatory tools to do so)
It’s ‘time for a reality check’ on the realistic prospects of progress towards the EU’s ambitious hydrogen goals, according to the European Court of Auditors’ (ECA) evaluation of the EU’s renewable hydrogen strategy.1 The same message is echoed in some recent assessments within member states, for example by… Read More
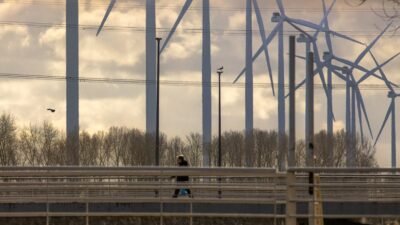
Financing the green transition: can private capital bridge the gap?
The green transition isn’t just about switching from fossil fuels to renewable or zero-carbon sources—it also requires smarter, more efficient use of energy. By harnessing technology, improving energy efficiency, and generating power closer to where it’s consumed, we can cut both costs and carbon emissions. In this episode of Top… Read More